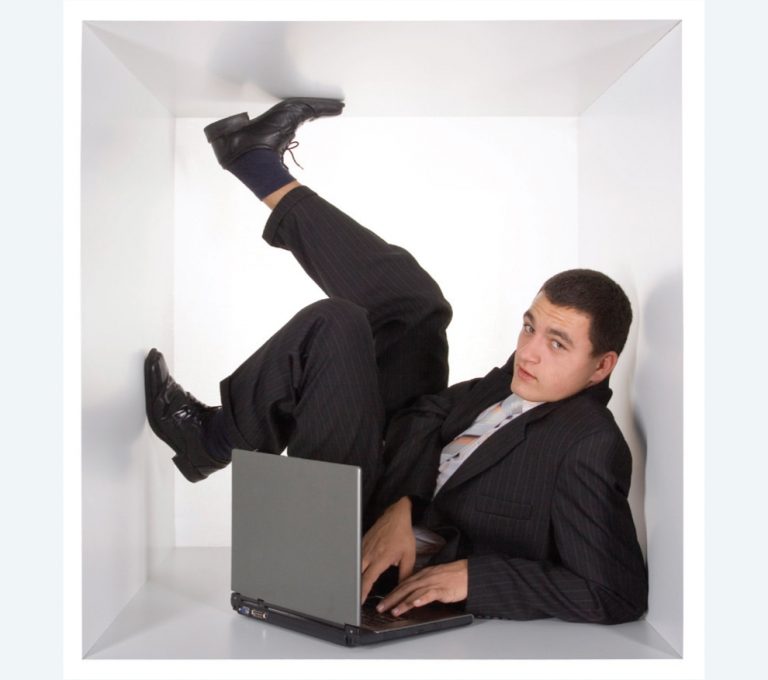
Using the Solvency II concept of “actuary-in-a-box” as a foil, Don Mango’s recent Explorations column (“Actuary-In-the-Box,” Actuarial Review, January-February 2014) provides a vigorous—and welcome—argument for the primacy of judgment in actuarial work. Along the way, Don points out that our profession seems to have a PR problem: For many people, actuarial calls to mind not-so-glorified number crunching—rote, mechanical and formula-driven. The notion of actuary-in-a-box (the technical term is re-reserving) abets this perception. It calls for a capital modeling team to encode the process by which an actuarial best estimate (ABE) of simulated future losses is arrived at. The unsettling implication would seem to be that loss reserving work is indeed mechanical in the way that popular imagination suggests.
Hopefully, few readers of this column believe that a purely formulaic approach to estimating future liabilities—one that dispenses with the need for professional judgment—will be forthcoming any time soon. As Don also points out, there is however a venerable school of decision science going by the name of “Clinical versus Actuarial Judgment” that seems to relegate actuarial work to the realm of rote, formulaic thinking. The field compares two modes of interpreting data. Clinical judgment involves processing and combining information in one’s head; the actuarial method dispenses with human judgment in favor of statistical tables or regression equations that encode empirical relationships and correlations. Once again, actuarial science seems to be identified with algorithmic decision-making that is free of the need for professional judgment. But contrary to appearances, this school of decision science in fact implies the opposite of this popular misconception. The actuarial work of building, criticizing and interpreting models is, has been and always will be infused with human judgment. And, far from entering an age where actuaries can be replaced by algorithms, we are entering an age in which these skills are needed more than ever.
The University of Minnesota psychology professor Paul Meehl initiated the field with the 1954 publication of what he later called his “disturbing little book,” Clinical versus Statistical Prediction. The studies Meehl discussed in this book, together with a cottage industry of studies that followed, overwhelmingly point to the conclusions that mechanically calculated scoring model predictions outperform those based on expert professional judgment. It turns out that the human mind is bad at aggregating information when making judgments concerning uncertain outcomes. Surprisingly, this is true even of highly trained experts seasoned with many years of professional experience.
Examples are not hard to come by. Predictive scoring models prove more accurate than clinicians’ diagnoses. They outperform admissions university officers’ judgments in predicting future academic success. They outperform the unaided judgment of expert underwriters at selecting and pricing insurance risks. In fact, they can even perform a limited, though reliable, form of marriage counseling: The late Robyn Dawes found that a particularly simple actuarial scoring model (subtract the frequency of quarrels from the frequency of love-making) is highly predictive of couples’ ratings of their marital happiness. The eminent psychologists Richard Nesbitt and Lee Ross drove the point home stating, “Human judges are not merely worse than optimal regression equations; they are worse than almost any regression equation.”
The actuarial work of building, criticizing and interpreting models is, has been and always will be infused with human judgment.
No matter how familiar these results become, they remain deeply unintuitive. To a statistically minded person surveying the evidence, it might seem plausible that, for example, a regression model could outperform a clinician’s judgment of the likelihood of the recurrence of a disease. But in the diagnostic heat of battle, it can be hard for clinicians or their patients to trust a model score over nuanced expert judgment. Subsequent findings shed light on why this is. Daniel Kahneman, the Nobel Prize-winning father of behavioral economics, was influenced by Meehl in his younger days. In Thinking, Fast and Slow, Kahneman writes that one’s degree of confidence in a prediction tends not to be based on a reasoned assessment of the predictive power of the evidence at hand. Rather, confidence is a feeling determined by the “narrative coherence” of the story, even when it is woven around sparse data. No wonder actuarial models run circles around expert judgment: Our confidence is increased by narrative details that, from a statistical point of view, are pure noise! Kahneman’s point also helps explain both the well-known phenomenon of overconfidence bias (we tend to believe our own narratives) as well as what Kahneman calls “the hostility to algorithms” (predictive model scores offer little in the way of narrative coherence).
The science of clinical versus actuarial prediction takes on added relevance now that business analytics and data science have entered the mainstream. Today it is fashionable to attribute the power of business analytics to big data: tweets, transactions, web-clicks and all the rest. But many business analytics projects owe their success less to the volumes, varieties and velocities of data involved than to the fact that they serve as correctives to the limitations of expert judgments. This, as Michael Lewis himself has recently acknowledged, is an implication of the book and movie Moneyball, and it also helps explain why scoring models outperform the unaided judgment of insurance professionals responsible for underwriting complex risks, judging instances of fraud or premium leakage, hiring agents or adjusting claims.
Returning to the initial question, do the Meehl school findings provide aid and comfort to those wishing to characterize actuarial work as routine or formulaic in nature? No. To paraphrase Albert Einstein, statistical models are “free creations of the human mind.” Meehl and his followers established that models are surprisingly effective essentially because the alternative—weighing information in one’s head—is so unreliable. But it does not follow that the models themselves can be algorithmically generated.
Barring radical breakthroughs in artificial intelligence, judgment will remain indispensable to the process of data analysis. There is no reliable way to algorithmically outsource the tasks of evaluating alternate model forms, selecting variables and blending qualitative background knowledge with incomplete or limited datasets. And this is doubly true of implementing (acting upon) model indications. Often even the best possible model is based on incomplete information. Furthermore, as Nassim Taleb discusses and loss reserving actuaries are keenly aware, there is never a guarantee that the data is complete or that historical patterns will carry into the future. Critical judgment is therefore indispensable in evaluating model risk: the possibility that a model indication could mislead in a particular situation. Judgment is therefore also needed to counterbalance the limitations of models with the case-specific knowledge of human experts.
The lesson of Meehl’s work is not that expert judgment is outmoded; rather that judgment is best directed away from the process of making case-by-case decisions in one’s head and toward the construction of models that weigh evidence so that these decisions can be made more consistently, accurately and economically. Actuarial judgment, in the sense of the type of professional judgment needed to be an effective data scientist, is essential to this process. Decision-making is central to all business, and the implication of Meehl’s work is that there is virtually no limit to the applicability of predictive models to improve decision-making. Actuarial judgment is entering its heyday.
James Guszcza, FCAS, is senior fellow at the Deloitte Analytics Institute in Singapore.