Actuarial Expertise
Explorations
Related Articles
Professional Insight
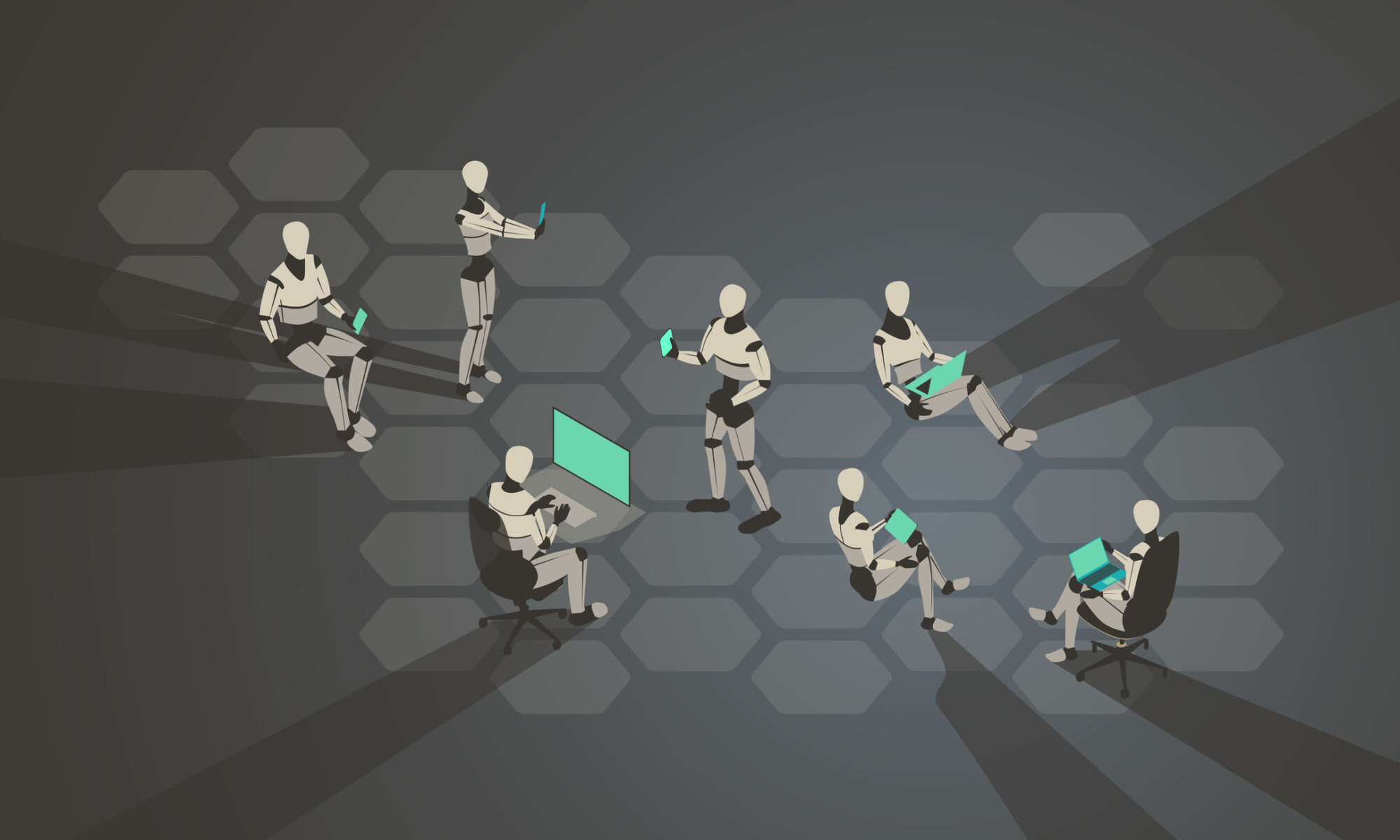
Agentic AI: Your New Actuarial Coworker
Professional Insight
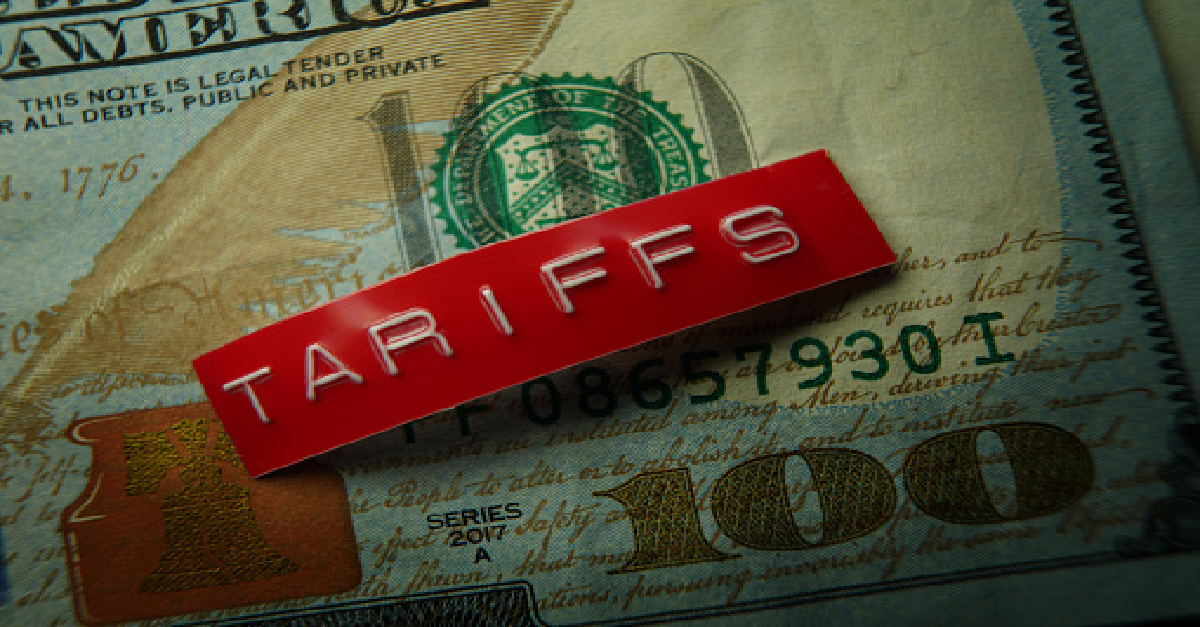
Tariffs Return: How Will They Impact Insurance?
Professional Insight
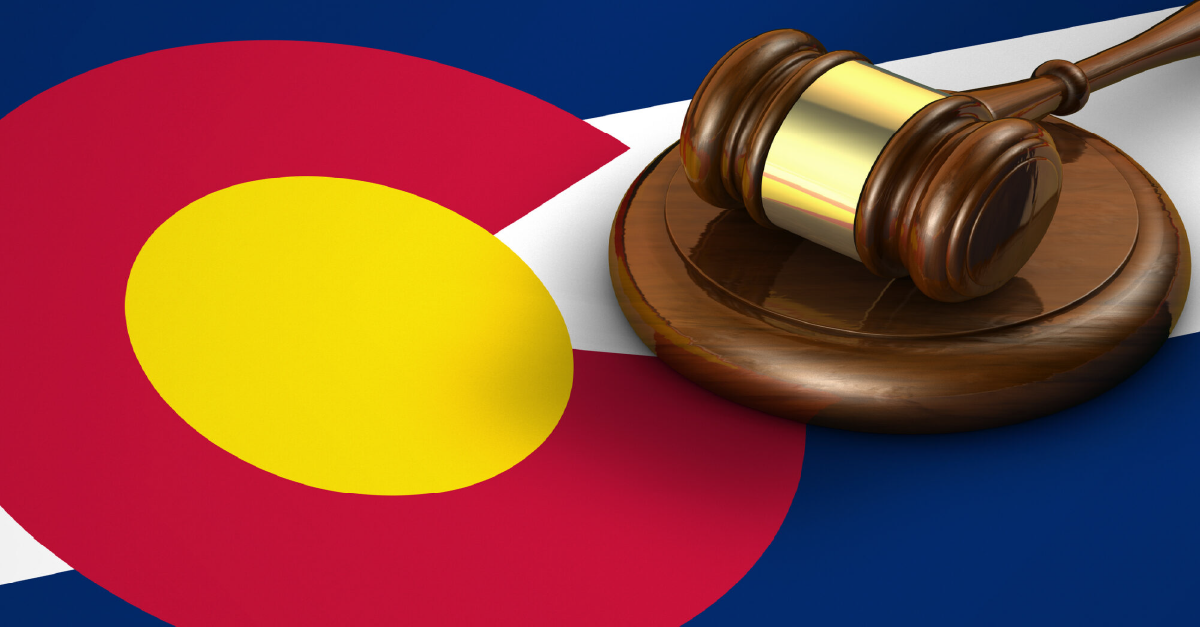