Federal Agency Aims to Manage or Reduce the Risk of Bias in Artificial Intelligence Systems
While the National Association of Insurance Commissioners (NAIC) has artificial intelligence (AI) resources directed specifically at insurance companies, its latest model bulletin also refers to the U.S. Department of Commerce’s National Institute of Standards and Technology (NIST) as a valuable resource regarding bias in artificial intelligence (AI) to numerous commercial and scientific entities.[1]
Like many working in insurance, the federal agency expresses concern that artificial intelligence systems (AIS), defined as a “machine-based system that can … generate outputs such as predictions, recommendations, … [that are] influencing decisions” can “potentially increase the speed and scale of biases and perpetuate and amplify harms to individuals, groups, communities, organizations, and society.”[2]
The NAIC on AI
The NAIC’s 2020 Principles on Artificial Intelligence[3] recommends that insurance professionals should promote AI, which includes data processing systems that perform human-like functions such as reasoning, learning and self-improvement, and considers machine learning as a subset of AI. The model bulletin states that AI should be fair and ethical, secure, safe and robust, accountable, compliant (with regulations) and transparent. The principles also recommend avoiding proxy discrimination against protected classes.
In December 2023, the NAIC issued a model bulletin titled, “Use of Artificial Intelligence Systems by Insurers,”[4] to establish some “expectations as to how insurers will govern the development/acquisition and use of certain AI technologies.”
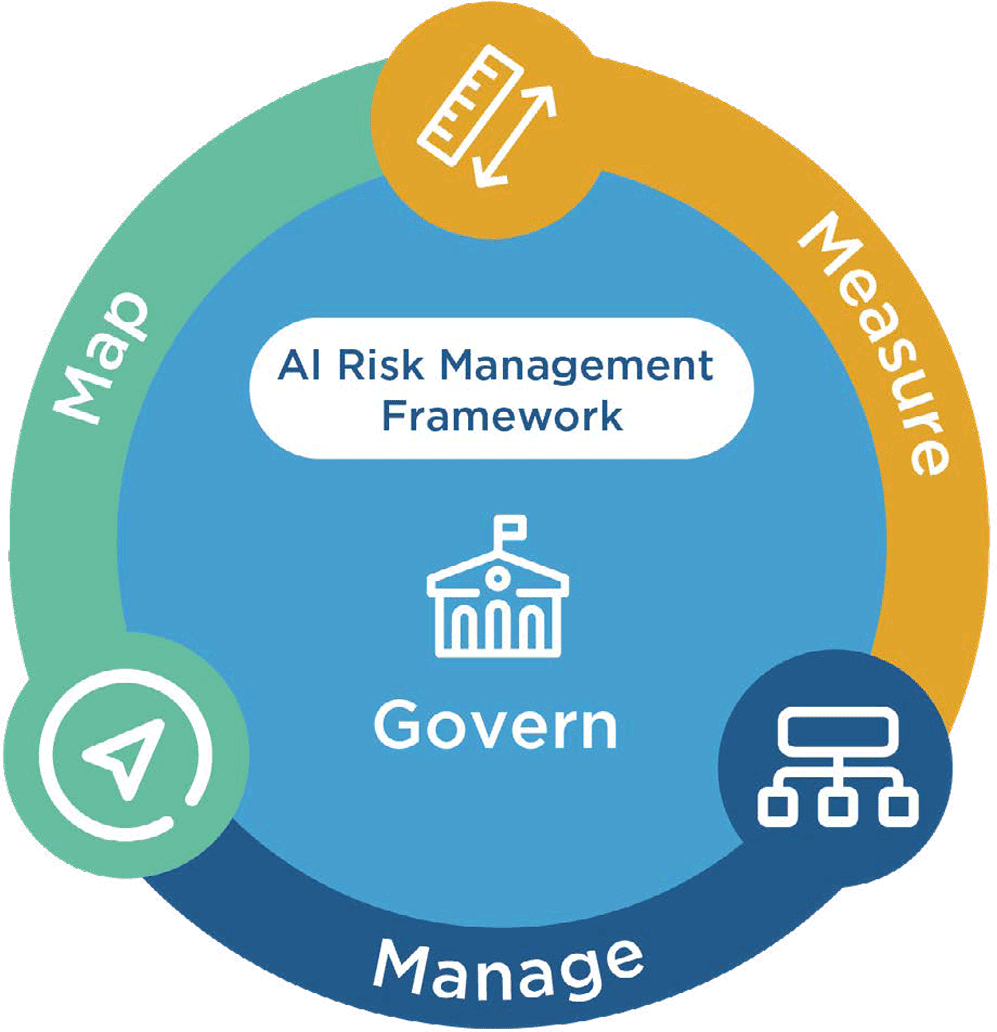
Further, the model bulletin’s Regulatory Guidance and Expectations section discusses the need for creating corporate guidance and internal controls specifically to mitigate the risk of adverse outcomes for consumers.
The model bulletin does not define bias, but it does offer that an insurer’s internal controls should include bias analysis and minimization. There was significant discussion about whether the word “bias” should be included in NAIC’s model bulletin. Some wanted the term removed or replaced with unfair discrimination or statistical bias, but ultimately, the word, “bias.” remained.[5] The model bulletin focuses on governance and risk management, including internal controls such as documentation of “the insurer’s risk identification, mitigation, and management framework … at each stage of the AI System life cycle.” Furthermore, it states that AIS risk management “should address the Insurer’s process for acquiring, using, or relying on (i) third-party data … and (ii) AI Systems developed by a third party.” The bulletin recommends NIST’s risk framework as one way for insurers to assess their AIS risk.
NIST
Since NIST is under the U.S. Department of Commerce, its focus is less industry-specific. In January 2023 under the direction of Congress with input from public and private sectors, NIST developed an AI risk management framework (AI RMF).[6] The AI RMF is intended to provide discussion and suggestions that will help to manage AI risks and develop trustworthy AI systems. The AI RMF states that to provide trustworthiness AIS must be valid and reliable, safe, secure and resilient, accountable and transparent, explainable and interpretable, privacy-enhanced and fair — with harmful bias managed.
Systemic bias refers to bias present in AI datasets, organizational norms, practices and processes across the AI lifecycle and the broader society.
NIST provides some good discussions around what bias means in Special Publication 1270,[7] titled “Towards a Standard for Identifying and Managing Bias in Artificial Intelligence.” But in AI RMF, the discussion is abbreviated, focusing on three major categories of AI bias to be managed: systemic, computational and statistical, and human-cognitive. (See Figure 1.)
Figure 1. Bias Types1
Systemic | Human-Cognitive | Statistical/Computational |
---|---|---|
Historical | Group | Processing, Validation |
Societal | Individual | Use and Interpretation |
Institutional | Selection and Sampling |
1 1 For more in-depth information on these bias types, see Figure 2 in NIST Special Publication 1270.
Systemic bias refers to bias present in AI datasets, organizational norms, practices and processes across the AI lifecycle and the broader society. Computational and statistical bias is bias present in AI datasets, algorithms and systematic errors due to non-representative samples. Human-cognitive bias can be individual or group bias and present in decision-making processes.
NIST AI RMF core is composed of four risk functions: govern, map, measure and manage. There is a playbook[8] developed to assist in working through the framework. A suggestion from the playbook about bias is to have the professionals evaluating results be independent from AI system developers to help “counter implicit biases such as groupthink or sunk cost fallacy,” which are forms of human-cognitive bias. The playbook recommends having a process for third parties to report potential concerns about potential biases in the AI system.
Future
NIST plans to continue to develop its risk framework, and it has a mandate to do so by Executive Order 14110: “Safe, Secure, and Trustworthy Development and Use of Artificial Intelligence.”[9] Several states have adopted NAIC’s model bulletin, while New York, as of this writing, was developing its own regulations. AI and bias will continue to be a subject worth monitoring, and those involved in all stages of an AI system life cycle may find these resources helpful.
Rebecca Armon, FCAS, MAAA, is a property-casualty actuary at the Texas Department of Insurance in Houston. She is also a member of the Actuarial Review Working Group.
[1] NIST, Artificial intelligence, https://www.nist.gov/artificial-intelligence.
[2] NIST, Artificial Intelligence Risk Management Framework (AI RMF 1.0) (nist.gov), https://www.nist.gov/artificial-intelligence.
[3] NAIC, Materials – Innovation and Technology (EX) Task Force, https://content.naic.org/sites/default/files/inline-files/AI%20principles%20as%20Adopted%20by%20the%20TF_0807.pdf.
[4] NAIC Model Bulletin: Use of Artificial Intelligence Systems by Insurers Model – Innovation, Cybersecurity, and Technology (H) Working Group (naic.org), https://content.naic.org/sites/default/files/inline-files/2023-12-4%20Model%20Bulletin_Adopted_0.pdf.
[5]NAIC Adopts Revised Model Bulletin on AI | Day Pitney.
[6]NIST Risk Management Framework Aims to Improve Trustworthiness of Artificial Intelligence | NIST.
[7]https://nvlpubs.nist.gov/NISTpubs/SpecialPublications/NIST.SP.1270.pdf.
[8] NIST AIRC Playbook, https://airc.nist.gov/AI_RMF_Knowledge_Base/Playbook.
[9]Federal Register, “Safe, Secure, and Trustworthy Development and Use of Artificial Intelligence,” E.O. 14110 of Oct 30, 2023.