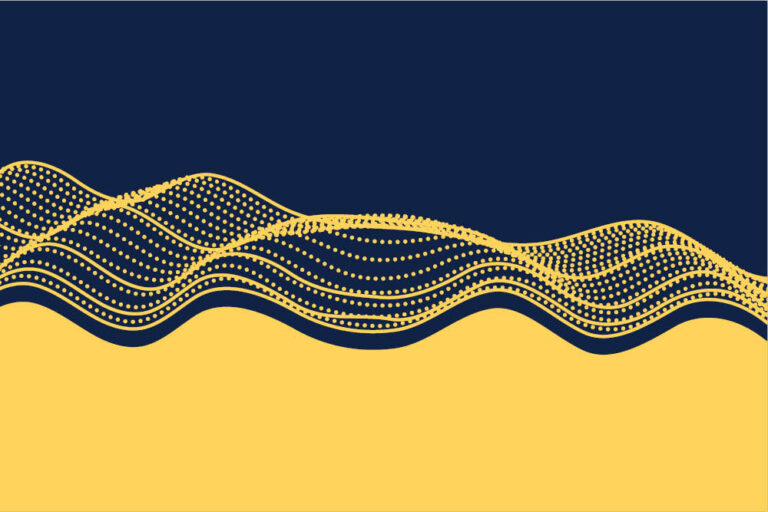
Always central to the work of actuaries, prediction methods have been transformed by increased computing power, access to high-dimensional data, and a rather surprising factor: the rise of the data science profession.
During a recent storyboarding session for AR, several of our writers anecdotally observed how more of their colleagues have been moving back and forth between actuarial and data science roles recently. This should come as little surprise. Since the inception of actuarial science, making predictions has been at the core of actuaries’ work. But their methods for making predictions have evolved in form and style, from simple algebraic formulae to the sophisticated machine learning algorithms used today. A surge in computing power and availability of higher dimensional data helped fuel the transformation — as did the growth of the data science profession.
In 2012 Harvard Business Review crowned data scientist “sexiest job of the 21st century,” just two years after the University of Chicago podcast CareerCast anointed actuary as the “best job in America.”1 During the decade since, as the role of data scientists matured, insurance companies realized the need to combine technical skills such as programming (a distinction of data scientists) with domain expertise (a very well-examined hallmark of actuaries) in order to drive value. As a result, neither profession’s value proposition has seemed quite as compelling on its own as when joined forces with the other.
As actuaries began to adopt analytics as part of their toolkit, they started to recognize certain limitations in their training concerning coding and machine learning. This is in contrast to data scientists who are more equipped for this technical heavy lifting — a byproduct of data science methods and technologies developing faster than actuarial educational curricula can keep up with.
Meanwhile, organizations began to reimagine the role of the data scientist from disestablishment to establishment.2 These developments in both career paths have increasingly fomented fluidity (perhaps even convergence) between the professions and a need for each party to raise and adapt its game.
Background and observed trends
The actuarial profession has existed since at least the 1700s, while the term data science did not enter the vernacular until the 1970s at the earliest. Both professions excel at analyzing data, forecasting future trends and offering data-driven solutions to the business. Actuaries are typically insurance professionals applying their skills to measure risk and uncertainty. Data scientists, on the other hand, tend to be generalists whose training in machine learning, artificial intelligence (AI) and big data technologies transcends industries or problem sets. Data scientists’ training may be specialized in knowledge areas such as modeling lifecycle and implementation tools, but even these specialties generalize across domains. Many years in an organization or industry may backfill domain expertise, but the interoperability of data scientists’ training arguably makes it easier for them to switch jobs between different industries throughout their career (especially during formative years). It also makes it more difficult to go deep within a domain — likely a reason, as noted in the introduction, some organizations are presently trying to institutionalize data science.
While actuaries’ domain-specificity makes them candidates to address some of the gaps data science leaves unfilled, the allure of a “sexier” career path is making actuarial resources scarcer.
While actuaries’ domain-specificity makes them candidates to address some of the gaps data science leaves unfilled, the allure of a “sexier” career path is making actuarial resources scarcer. Some challenges remain — a growing demand, higher-than-average entry salary and diverse job opportunities have fueled the growth of the data science job market in recent years. With higher entry-level salaries for data scientists at a median of $87k (compared to entry-level actuarial jobs at $65k based on Payscale) in 2024, an increasing number of recent actuarial grads have moved to data science jobs. However, over the long term actuarial jobs have higher median salaries. According to the U.S. Bureau of Labor Statistics (BLS), in May 2023 the median actuarial salary was $120K compared to $108K for data scientists — a reflection that greater STEM earning potential may come from going deep and specializing. Yet the disparity at entry-level positions adds risk to continuing to attract top talent into the profession.
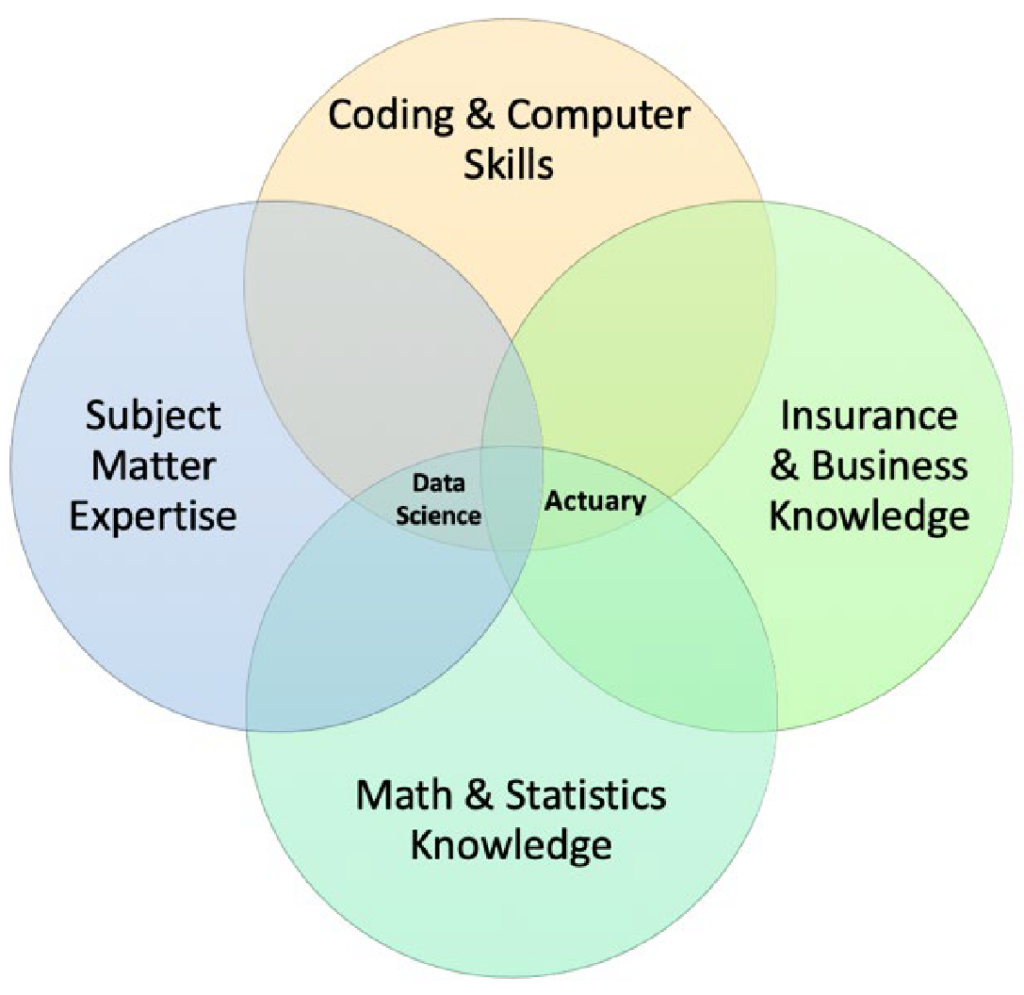
Figure 1. Respondents interested in increasing their proficiency
Despite entry level STEM professionals skewing a bit more data scientifically, more experienced actuaries by choice or necessity are doubling down on their domain expert bona fides. From a skills perspective, despite citing in the “CAS Actuarial Technology Survey” that actuaries are eager to learn more scripting tools such as R and Python, relatively few are doing so with the greatest barrier cited being a lack of time. These tools are considered part of the basic toolkit of a data scientist, creating a reliance on data scientists to complement actuaries in terms of coding skills and help plug the gap in knowledge or transfer it over.
Despite their industry specialist reputation, actuaries may be more adept at being STEM generalists than they realize. It’s common to have actuaries perform tasks outside of their areas of training and to rely on other experts to fill any gaps in knowledge or expertise. This is even institutionalized in ASOP No. 1, Section 2.11 (Reliance):
Actuaries frequently rely upon others for information and professional judgments that are pertinent to an assignment.
One could argue the territories seized by data scientists in recent years were lands actuaries never sought to inhabit in the first place given these standardized predispositions.
Challenges and opportunities
Actuaries have not ceded significant ground yet, with a job growth outlook of 22% (according to the BLS in 2023) but the concerning trends in entry-level employment do suggest they should consider expanding their borders — and they are doing so. In 2023 the CAS launched a new educational requirement with its online course Data Insurance Series Course: Introduction to Data and Analytics (DISC DA), further promoting the integration of data science and actuarial science in actuarial curricula. Starting in 2025, candidates will need to complete a predictive modeling project in order to achieve membership.
On the other hand, this revolution in analytics is an opportunity for actuaries to leverage advanced algorithms and predictive models more quickly to improve accuracy of predictions and to analyze large datasets more efficiently, so that they remain ahead of the game in a highly competitive market. This may help replenish actuaries’ “cool factor” in the entry-level STEM market. More broadly, actuaries can and will continue to outsource responsibilities to data scientists when situations call for it but can now be intentional about how and when to do so.
Despite their industry specialist reputation, actuaries may be more adept at being STEM generalists than they realize.
The times for actuaries to outsource will most likely still be matters of technical specification that are not domain-specific. To adapt new technologies and leverage the full potential of algorithms such as Generative Pre-training Transformers (GPTs), actuaries are faced with challenges as well as opportunities. An actuary’s technical skills often hit a time limit (per survey finding earlier), creating a need to rely on experts with deeper technical skills such as data scientists, data engineers and AI engineers. However, hand-off frictions can make this an inefficient exercise, creating a “Sophie’s Choice” for both professions of whether to invest time to upskill outside their sweet spot versus upskill their counterpart outside of theirs. This dynamic has thus blurred the job requirements of each profession, with assigned tasks and responsibilities that may seem overlapping — and, in some cases (as noted before), professionals electing to “cross over” to the other side.
On the bright side, a sensible and equitable division of labor has organically emerged out of the ambiguity. The fluidity between careers allows organizations to bring expertise from both sides to problems, facilitating better, faster decision making and adoption of new technologies. The fact that data scientists have greater career options outside of insurance makes it harder to retain data science talents in insurance over the long term and reinforces a reliance on actuaries to develop some of this expertise to ensure the acquired knowledge won’t be lost. In recent years, the role of a data scientist at different companies or institutions has evolved more from machine learning to machine learning operations, clarifying actuaries’ traditional roles in creating solutions and data scientists’ trademark facility with code.
While organic solutions have emerged for integrating data science into organizations, changes will continue coming quickly — and organizations need not wait for solutions to emerge on their own. The challenges discussed in this article raise some open-ended questions to the actuarial community:
- How can we better facilitate the collaboration and knowledge sharing between two professionals?
- What is the most efficient way to integrate data scientists into the current corporate structure?
- How can we maintain the knowledge continuity with talents coming and going over time?
Embracing the change
The actuarial profession will continue to evolve and to adapt to an ever-changing technological landscape. Relying on other professionals (including but not limited to data science) with different expertise is inevitable in a fast-evolving, modern world. To maximize the full potential of data science in insurance, this requires a commitment to acquiring new skills, developing actuarial education and more effective collaboration with other professionals. The data science career has also progressed throughout the years and will continue to change by adapting professional skills and market needs. Learning to collaborate and work with professionals with different skillsets and knowledge should be at the core of an actuary’s training. This shift will lead to communication skills continuously increasing in value. A more fluid career path offers deeper learning opportunities and a wider range of experiences beyond those acquired through formal training. Fluidity is an important element of becoming a data-driven culture and drive value for employers, yet it comes with challenges. Actuaries will need to understand both the opportunities and challenges to allow their employers to leverage the benefits of this fluidity between careers.
Yuhan Zhao, FCAS, is a senior actuarial manager with Aviva Insurance Company of Canada. She is news editor for the AR Working Group and a member of the Monograph Editorial Board. Sandra Maria Nawar is a manager for Intact Financial Corporation in Toronto. She is a member of the AR Working Group Writing Staff. Jim Weiss, FCAS, CSPA, is a vice president for Crum & Forster and is editor in chief for Actuarial Review.
1 https://www.cnbc.com/2010/01/06/The-Ten-Best-Jobs-in-America-2010.html.
2 https://hbr.org/2022/07/is-data-scientist-still-the-sexiest-job-of-the-21st-century.
References
- https://www.dwsimpson.com/2024/04/12/the-increasing-role-of-data-science-in-actuarial-work/.
- https://www.linkedin.com/pulse/transitioning-from-actuary-data-scientist-guide-andrew-chan-anazc/.
- https://www.linkedin.com/pulse/actuarial-science-vs-data-coaching-actuaries/.
- https://www.zdnet.com/article/data-scientists-still-the-sexiest-job-if-anyone-would-just-listen-to-them/.
- CAS Data and Insurance Series Courses | Casualty Actuarial Society (casact.org).
- Entry-Level Actuary Salary in 2024 | PayScale.
- Entry-Level Data Scientist Salary in 2024 | PayScale.
- https://hbr.org/2022/07/is-data-scientist-still-the-sexiest-job-of-the-21st-century.
- https://www.casact.org/sites/default/files/2022-03/CAS-RP_First_Annual_CAS_Actuarial_Technology_Survey.pdf.
- https://www.computerscience.org/resources/actuarial-vs-data-science/.
- https://www.bls.gov/oes/2023/may/oes152011.htm.
- https://www.bls.gov/ooh/math/data-scientists.htm.