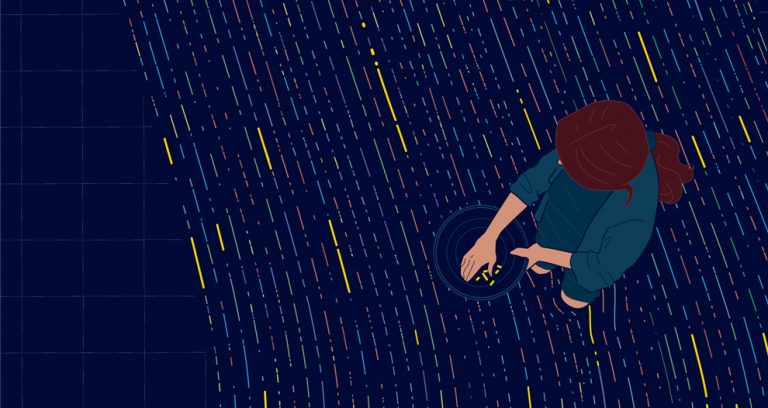
Data may be in abundance, but it’s not all valuable. Actuarial prospectors must sort through the morass to find the meaningful nuggets — and do it fast to keep up.
Predictive modeling is advancing far beyond its general linear modeling (GLM)-based roots. Thanks to the explosion of new data sources, technological innovation and advanced analytics, predictive modeling is promising solutions while being poised to disrupt the insurance company business model.
The solutions vary by each property-casualty insurance line. Generally, personal lines insurers are enjoying more opportunities for building proxies for human behavior beyond tried-and-true credit scoring. Faced with the boundless multiplicity of potentially predictive factors, commercial lines carriers are determining risk by attaining deeper client knowledge while focusing on uses beyond pricing including claims management, underwriting and premium auditing.
To reveal the latest trends in predictive modeling, Actuarial Review is presenting a three-part series. This first article focuses on data: the energy that drives the models. Part II will consider the vehicle for analyzing data: the models. Part III will take a closer look at the implications of modeling going forward, including decision-making, regulatory considerations and loss mitigation.
The Quest for Data
From the potential of big data to the internet of things (IoT), data buzz abounds in the property-casualty industry. The widening stream of data availability has created a rush to find competitive data gold, requiring actuaries to sift through the electronic morass to find truly valuable indicators that will meaningfully answer questions.
The reason is simple. “The predictive models get better with new data sources,” said Christopher Monsour, vice president of analytics at CNA.
The data influx derives primarily from external data offered by vendors, sources said. “More companies are offering more information,” said Roosevelt C. Mosley, principal and consulting actuary for Pinnacle Actuarial Resources. “It seems to keep going and at some point you think it will level off, but from my perspective it hasn’t.”
Some vendors are expanding their products and tools while others are new entrants into the insurance market. Procuring and analyzing data are expensive but necessary, Mosley said. “The goal in this arms race is to find things that allow you to get ahead of your competitors or keep up with them, and it creates the dichotomy of the haves and have nots,” he explained.
Big companies have an advantage in today’s data and analytics arms race, said Stephen J. Mildenhall, who recently left his post as global CEO of Analytics for Aon to join the faculty at St. Johns University’s risk management and insurance department. Larger insurers “have more data and can invest more in statisticians and modelers to uncover relationships in that data,” he added.
What kind of data actuaries need depends on several variables. These include: the question being asked, the property-casualty line and the type of model. Data and the kind of model being used should work in tandem along with other considerations, said Serhat Guven, Willis Towers Watson’s P&C sales practice leader for the Americas. “(Without) thinking about the data, the modeling layer becomes worthless,” he noted.
There are also legal and regulatory restrictions to recognize along with ensuring data is being used in its proper context, Mildenhall said. “There is a real danger, if you do not … understand the data elements you use, you are going to get bad readings coming out,” he added. He cited Google’s attempt to predict flu outbreaks based on web searches, which worked until the company changed its algorithms.
Finding Reliable Proxies
Since personal lines insurers cover people and their property, one goal is to find relevant factors that indicate the behavioral risk of current and potential customers. In particular, much potentially relevant behavioral information for personal lines emanates from the “data breadcrumbs” people leave through internet searches, social media participation, digital wearables and mobile devices, as well as connected cars and dwellings, said Jim Guszcza, U.S. chief data scientist at Deloitte Consulting.
Using data footprints from multiple sources, such as online searching and shopping, for consumer marketing is a common practice for organizations, including insurers. What’s different now is that insurers are finding some of this information helpful for building proxies predictive of potential behavior.
Credit scoring remains the best example of a reliable proxy that predicts how people are likely to behave by correlating biological and psychobehavior1 with risk taking. It provides the proof that “a man drives as he lives,” a conclusion from a 1949 research study.2
There are deep reasons why credit scoring works, including human brain chemistry and neurotransmitters, which is why impulse control with money and driving often have the same underpinnings, Mildenhall said. “People have reasonably fixed personalities to tease out,” he explained, and since their behaviors tend to be immutable, additional information provides a better picture of risk.
The ultimate goal for personal lines predictive modeling is to find data that provides another useful psychological proxy like credit scoring. “If someone discovered the next credit score, (it would) get locked up in a vault and no one is going to talk about it,” Mildenhall said. “It is a huge competitive advantage if you can figure that out.”
Consumer information such as magazine subscriptions and purchases at home improvement retailers can predict if a homeowner is committed to home maintenance and how that reflects risk. Insurers and vendors are already looking into these types of relationships, Mildenhall said.
Using credit scores to select and price personal home and auto is a familiar example of the predictive power of nontraditional behavioral data sources. Supermarket loyalty card data can be similarly predictive of people less likely to file claims.3 More recently, the sort of lifestyle data traditionally used for target marketing has been repurposed to help infer individuals’ health risks4 — an application of use to life insurers and healthcare providers. As an added bonus, healthy consumers also are more likely to physically recover faster from accidents, which reduces medical costs.
“If someone discovered the next credit score, (it would) get locked up in a vault and no one is going to talk about it,” Mildenhall said.
While finding useful data for reliable proxies is a critical piece of the predictability puzzle, telematics offers something more novel: actual driver data. Considered by many to be the first true foray into the IoT, large insurers have enjoyed the competitive edge telematics can provide.
However, “the plug-in solution is on its way out,” Mosley said, because smart phone tracking apps are proven to be just as effective as black boxes. “That will help a lot of small companies that could not pay for devices,” he explained. Eventually, apps will become obsolete in the advent of imbedded devices installed by vehicle manufacturers and semiautonomous or autonomous cars, he added.
Guszcza said that while automobile telematics data is “particularly relevant for predicting auto accident frequency and severity,” any behavioral data gleaned from nontraditional sources is potentially relevant for inferring various types of insurance risk behaviors.
Meanwhile, insurers are still learning how to benefit from all the telematics data they are or could be collecting, Mildenhall said, noting the technical problems with acquiring large amounts of detailed information on a real-time basis. Further, he noted, “underwriters and regulators are not happy with black box models in part because it is hard to prove they do not discriminate in some unwitting way.”
In addition, usage-based insurance “is not something consumers are clamoring for and I don’t see that changing relatively soon,” added Mosley, due to privacy concerns and lack of a compelling value proposition. Consumer privacy concerns, of course, are not limited to telematics data. As the volumes and level of detail of consumer data continue to increase, insurers must be careful to use the information ethically, said Guszcza, who desires a greater industry conversation about data use.
Beyond human behavior data, personal auto insurers can access other useful information. For example, more data is available about the vehicles themselves through various vendors including CarFax, which can also fine-tune ratings, Mosley said.
Besides external sources, some insurers are discovering additional policyholder data that is useful for predictive modeling, Mosley noted, such as the date of policy purchase. Advance purchasing, like credit scoring, indicates responsible customers who also tend to have a more favorable loss history, he added. As a result, some companies are rewarding customers who buy policies seven days before the policy date with an advanced quote discount.
On the commercial side, there is greater accessibility of text data from claims adjuster notes, Monsour said.
Commercial Cues
For commercial lines insurance, data availability varies greatly by line. When asked where predictive modeling will have the most impact in commercial lines, Guven cited workers’ compensation, commercial auto, commercial property and business owners policies (BOP).
“The more data, the more common the risk, the more valuable our predictive modeling becomes,” he said. For unique specialty lines, Guven explained, insurers do not have sufficient data for predictive modeling.
Detailed, granular data is already widely available on crime rates and weather; but looking forward, risk assessment for engineered buildings could be based on architectural and building plans, Mildenhall said. “By feeding plans into a computer, insurers can know the location of every nut and bolt and could use that for catastrophe risk assessment.”
Commercial lines require more qualitative information than personal lines because there are more variables to consider. A company’s nature of operations and services being offered are two examples, Monsour said, because two companies can be considered retailers, but one that sells equipment has different risks than one that installs it. Other qualitative information includes management quality, liability chain/supply chain issues, strength of a company’s hold-harmless provisions, and other risk transfer provisions such as contracts with subcontractors, he added.
Another major consideration is that while personal lines actuaries can rely on the fact that people generally do not change, commercial lines actuaries have to keep up with the dynamic nature of organizations, Mildenhall said, due to new management priorities, growth, location and other factors. “The company you measure one year can be different from what you measure next year,” he explained.
The good news is that more useful data has become available for commercial lines predictive modeling, Monsour said. However, it requires very careful examination. In his experience with commercial lines data, “Many vendors do not have useful data and you have to evaluate them quickly to sort the wheat from the chaff.” Many data providers are new to the insurance market, he explained, and might not have necessary historical data. It is critical, he advised, to determine how much coverage the vendor offers and how many customers the data covers, and to review a sample.
Vendors also offer similar types of data, but it is difficult to evaluate accuracy when cross comparisons of information among vendors is not possible, according to Monsour. Further, customers can have several company names or “doing-business-as” (DBA) names. “The data has to match the customers,” he added.
While vendors are actively trying to sell external data to commercial insurers, some of the potentially best predictive information belongs to customers who are unwilling or unable to share it. From an IoT perspective, information from cameras and sensors located on many commercial properties would be helpful for pricing commercial package coverage, Monsour said. “The hard thing is getting permission to access the data,” he noted.
This also holds true for telematics. While personal lines actuaries are benefitting from actual driving behavior information, commercial auto insurers are struggling to collect the same material about employee drivers. “The story of telematics is the fleet managers have the data but nobody wants the insurance companies to have it,” Monsour said. To solve this problem, some insurers are offering discounts.
Another difficulty is that commercial lines insurers face limitations in using the same kind of personal information as personal lines can when the same person, in effect, is being covered. (This issue will be further discussed in part III of this series.) Employers usually take the privacy of their employees very seriously and would likely have a lot more to lose than to gain, Mildenhall said. There are also employment laws and state insurance regulations that can limit what commercial insurers can use.
Future Data
While actuaries are looking forward to the IoT for its data potential, many sources believe realizing the data for modeling purposes remains years away.
Already, forward-moving insurers are exploring the potential of IoT information in the home to detect problems with water leaks, carbon monoxide and other causes that lead to claims. “The most I have seen up to this point are companies developing partnerships and/or other facilities to take advantage of this kind of information,” Mosley said.
Some insurers are also offering discounts to encourage smart home detection. Liberty Mutual’s Smart Home Discount Program rewards customers with savings for adopting self-monitored and professionally monitored protection devices for theft, fire and water; and the discount doubles if the customer allows data sharing for verification purposes. State Farm also offers discounts for certain smart home systems.
Even so, since older generations are more privacy-oriented, Mildenhall said, it would take many years for homeowners to install home IoT devices.
Already, forward-moving insurers are exploring the potential of IoT information in the home to detect problems with water leaks, carbon monoxide and other causes that lead to claims.
For commercial lines, Monsour said, insurers will be spending the next decade determining how to integrate coverage with IoT. For example, if a commercial insurer could integrate with a fleet management service — which optimizes factors such as routes and gas mileage — they would have access to a huge amount of information that is otherwise difficult to obtain, he explained.
“Similarly,” he said, “integrating with a security company that has cameras in a warehouse would allow the insurer to use the cameras for other things, like detecting fire hazards on an ongoing basis and warning about them,” or ensuring that the owner is having sidewalks cleared of snow.
Monsour is optimistic that IoT will provide additional useful data for commercial property coverage. Guven, however, is less hopeful about the predictability of sensor technology for it or homeowners policies.
Mosley sees great potential from drones that are currently taking photographs of property for purposes of claims adjustment. “These drones are collecting a ton of data. While companies might not be using it at the moment, I think it is information we are going to figure out how to use somehow — and it is going to become much more valuable,” he added.
Sources are also hoping that converting text to data will also unearth more predictive power. “A lot of the most interesting sources about businesses,” Monsour said, “have a lot of text. How do you best leverage that kind of information?”
Conclusion
In the quest to find predictive correlations within data, actuaries are finding that reliable, rich and contextual data that is useful for predictive modeling is becoming more available in some areas. However, data scarcity continues to leave important questions about risk unanswered, especially for commercial lines.
Looking forward, technological advancement, the continual expansion of data collection, potential revelations through IoT, consumer privacy concerns and regulatory determinations will greatly affect both the availability and usability of future data.
Ultimately, however, the models determine the value of data. The next article, “Modeling Predictability,” will delve into the latest models, their purposes and applications beyond rating and pricing.
Annmarie Geddes Baribeau has been covering actuarial topics for more than 25 years. Her blog can be found at http://annmariecommunicatesinsurance.com.
1 Brockett, Patrick L., and LindaGolden, “Biological and Psychobehavioral Correlates of Credit Scores and Automobile Insurance Losses: Toward an Explication of Why Credit Scoring Works,” The Journal of Risk and Insurance 74:1, 2007, pp. 23-63.
2 Tillman, W.A., and G.E. Hobbs, “The Accident-Prone Automobile Driver: A Study of the Psychiatric and Social Background,” The American Journal of Psychiatry 106, 1949, pp. 321-331.