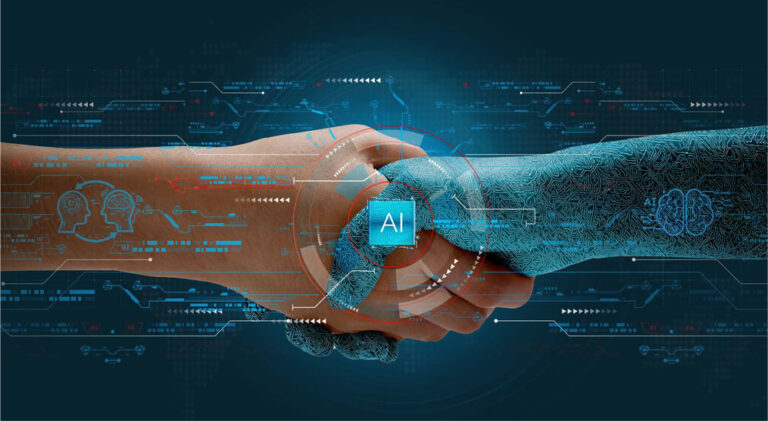
James Guszcza became an actuary because he wanted to do data science work, even though that field didn’t exactly exist. Data science was evolving, so it didn’t have a name yet. In this Annual Meeting session, “Professionalizing Artificial Intelligence: Lessons from Actuarial Science,” Guszcza looks at the diverse skills and perspectives that actuaries apply to a wide variety of business problems and presents us with a framework for what he proposes is another new job category: hybrid intelligence.
Artificial intelligence (AI) can be viewed from three perspectives, which are not as diametrically opposed as they may at first appear:
- AI is the new electricity, meaning it is a general-purpose technology.
- AI is an “intellectual wildcard,” meaning an all-encompassing term for any kind of emerging technology, beyond the boundaries of scope and consequences.
- AI is an ideology, meaning the application of machine learning can advance a wider goal to benefit humanity.
Effective and ethical AI needs human-centered design.
The third perspective is rooted in the history of AI. The founding fathers of AI first gathered at the 1956 Dartmouth Conference. They thought they could solve intelligence by 2001. This timeline assumed that human intelligence was doing calculations and drawing logical conclusions. This is what is considered today to be First Wave AI. Second Wave AI is based on machine learning, statistical pattern recognition in large data sets and especially deep learning.
We often hear concerns that AI will evolve into something beyond human control — perhaps the type of fears portrayed in a science fiction movie. This is often driven by the sloppy language that we wrap around the technology and can lead to “artificial stupidity.” Guszcza proposes that we break artificial stupidity into two problems:
- The first mile problem: Training data isn’t given; it must be designed. Train an algorithm on only one type of risk and it won’t recognize others. Additionally, sometimes there are not enough edge cases in the data to allow the algorithm to adequately predict these possibilities. Next, you must create a proxy variable for the outcome being predicted. But certain social constructions do not have objective definitions — race, gender, “healthy patient,” “good employee,” etc. What one person considers to be a “good employee” may be very different from another person’s definition.
- The last mile problem: We care about outcomes, not algorithmic outputs. A model can perform very well and accurately segment good risks from bad risks, but one must recognize the human element of implementation. If underwriters are only discounting the good risks but not surcharging the risks that the model identifies as bad risks, the modeling effort has not achieved its goal. The key is to build good models and integrate them into smart workflows, recognizing the importance of both the algorithmic output as well as the human element.
Effective and ethical AI needs human-centered design. Guszcza recalled a quote from Richard Nesbett and Lee Ross, psychologists at Stanford: “Human judges are not merely worse than optimal regression equations. They are worse than almost any regression equation.” The places most susceptible to human bias are where algorithms can reduce bias. And yet, today’s algorithms can’t replace experts. The first mile problem tells us that humans must make the decision about what data should be used to train the model because only humans can tell the difference between appearance and reality. The second mile problem tells us that the implementation of any modeling solution will rely on an interaction with a human element. Humans working together with AI creates something Guszcza refers to as a “diversity bonus” or a “collective intelligence” in which the outcome is superior to that which would be produced by either on their own.
Hybrid intelligence development is the intersection of the following fields:
- Statistics and machine learning — grounded in computation and statistics.
- Participatory design — multiple stakeholders and domain experts providing important context and facilitating ethical deliberations.
- Behavioral sciences — recognition of human behavior, change management, and organizational design.
Guszcza does not consider actuarial science to be “applied math,” just as he would not call hybrid intelligence development “applied computer science.” But rather, he says, each can be viewed as “computational social sciences.” This field, comprised of learned professionals, can optimize how humans interact with algorithms to achieve optimal societal outcomes that cannot be achieved through regulation alone. Designing the human-machine interaction processes is an essential component.
Erin Olson, FCAS, is a member of the Actuarial Review Working Group and the new CAS VP-Marketing Communications. With 21 years in the actuarial field, Olson currently leads a group of decision science analysts, supporting property claims at USAA.