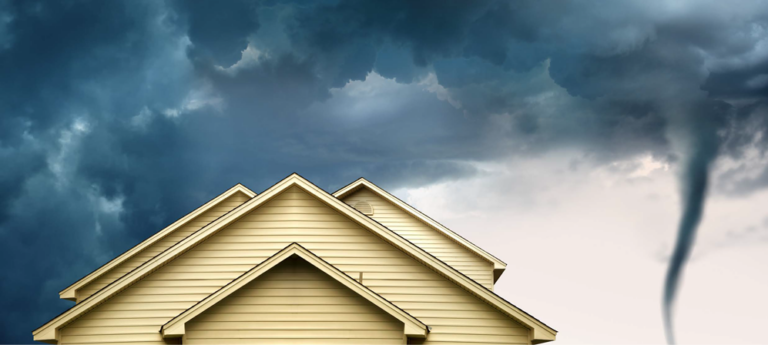
Over the past seven years, I have worked in homeowners pricing for two different companies. In that time, I had the opportunity to work with rating algorithms for all perils combined and by peril. For those not familiar with this topic, “by peril rating” means that instead of calculating just one overall homeowner premium (the “all perils combined” approach), smaller premiums for constituent perils (e.g., fire, water, hail) are calculated first and then these separate peril premiums are added together to arrive at the total homeowner premium.
Mistakes happen . . . Mistakes eventually get found, and the ones that linger longer are more costly to address.
An overwhelming majority of my business partners would passionately insist that by peril rating is the superior approach; two separate research areas that I have partnered with have shown double-lift charts supporting the assertion that by peril rating allows actuaries to estimate losses more accurately. More accurate estimated losses will produce more accurate premiums, which in turn allows insurance companies to charge lower premiums to win the better risks and charge higher (but accurate) premiums to write relatively less of the worse risks.
This all sounds great … in theory.
Nothing is ever so simple, however. In addition to providing a necessary public service and protecting individuals and families from financial ruin, insurance companies are keenly interested in making a fair and reasonable profit. A very important question that must be carefully considered is:
What is the financial impact of utilizing a by peril rating algorithm over a simpler all perils combined rating algorithm?
As actuaries, we have been extensively trained to use multiple techniques to help us determine rates that are “not inadequate, excessive or unfairly discriminatory.” For the sake of argument, let’s trust the results of my partners in the research areas at both companies and assume that by peril rating allows us to charge more accurate premiums and mitigate subsidies introduced by utilizing an all perils combined rating algorithm. Assuming we all agree that by peril rating is more accurate, then why would an insurer use an all perils combined approach?
This brings us back to our very important financial impact question above. A financial impact is going to have costs and benefits. Here is where the analysis gets a bit complicated.
The cost side of the equation is more straight forward. On the cost side, there is a much more substantial workload involved in creating, monitoring and maintaining a by peril rating algorithm versus a much simpler all perils combined rating algorithm. If the by peril rating plan has eight perils, then pricing actuaries will need to make eight times the assumptions than they otherwise would. There will be eight times as many numbers to check and implement. In terms of monitoring results (i.e., indications), cutting the data by state and peril can result in extremely thin data for most small-to-medium insurance carriers. When all is said and done, pricing actuaries wind up reviewing thinner data and are forced to make more assumptions based on the thinner data. Unfortunately, despite the substantially larger work efforts involved in arriving at a reliable indication at a state and peril level, there is a material amount of uncertainty in the final indications.
Monitoring uncertainty aside, another potential pitfall is that a more complicated premium calculation leads to a higher chance for errors. Mistakes happen. In most cases, it is easier to detect and then quickly address an error in an all perils combined rating algorithm than it is for a by peril rating algorithm. As an example, if one of your rating factors is off by 10% in an all perils combined rating algorithm, that 10% error will be more apparent than if the fire peril territory factor is off by 10%, which might only impact the final premium by 2%. Mistakes eventually get found, and the ones that linger longer are more costly to address.
Overall, the additional labor associated with employing a by peril rating algorithm is likely to be several additional headcounts in the insurance organization, which likely equates to a few hundred thousand dollars annually. (Individual company results will vary.)
The benefit side of the equation is far more difficult to pin down. To accurately ascertain the net benefit of by peril rating, we would need answers to the following questions:
- How many better customers are acquired due to lower premiums from by peril rating?
- For the better customers that are retained, how much less premium is collected due to charging less because of by peril rating?
- How many of the worse customers are lost (that would have been written) due to having higher premiums from by peril rating?
- For the worse customers retained, how much more premium is collected due to charging more because of by peril rating?
As you might imagine, obtaining definitive and clear answers to the questions above is impractical.
However, despite obstacles thwarting our quantitative tool kit, we can still use our imagination and judgement and qualitatively assess the benefit side. If the premiums charged are now more accurate, the insurance company will now be able to improve the quality of their book of business since they will be more competitive with the better risks and less competitive with the worse risks.
On the cost side, we estimate that the annual cost delta of the by peril rating algorithm will be equal to several full-time equivalents, which will cost a few hundred thousand dollars extra each year. Switching from an all perils combined rating algorithm to a by peril rating algorithm makes sense only if over the long term we believe the by peril book would out-perform the incumbent all perils combined book by an amount greater than the incremental cost of running the by peril rating plan.
This analysis is far from a straightforward exercise, but that is the joy of our profession.
A typical homeowners profit provision is generally between 8% and 12%. For this back-of-the-envelope exercise, let’s assume premiums are priced with a 10% underwriting margin. Let’s also apply this analysis to a $100 million-book of business. That is to say, the expected annual underwriting profit is $10 million. Do we believe that the by peril rating algorithm will over the long run consistently generate roughly half a point (i.e., +$500K/$100M) of additional margin to cover the added intrinsic costs of executing by peril rating?
To answer this question, we review the four questions above.
Notice that question #1 will offset question #3 to some degree. The insurer will gain some better new business at an adequate return and lose some worse existing business at a less adequate return. So long as the insurer is gaining enough of the better business to cover the lost worse business, all is well.
Next note that question #2 will to some degree offset question #4. The insurer will lose premiums from the retained better risks as they will now be charged the lower more accurate premiums; the insurer will gain premiums from the retained worse risks. Depending on the mix of business and attrition rate in each group, this could result in a net benefit or cost to the insurer.
This analysis is far from a straight-forward exercise, but that is the joy of our profession. For what it’s worth, in response to the question as to whether insurers should switch from relying upon a simpler all perils combined rating algorithm to a by peril rating algorithm, I can confidently assert that over the long run, it might be beneficial.
Maybe.
Rob Kahn, FCAS, is a pricing manager for Horace Mann Insurance and a member of the Actuarial Review Working Group.