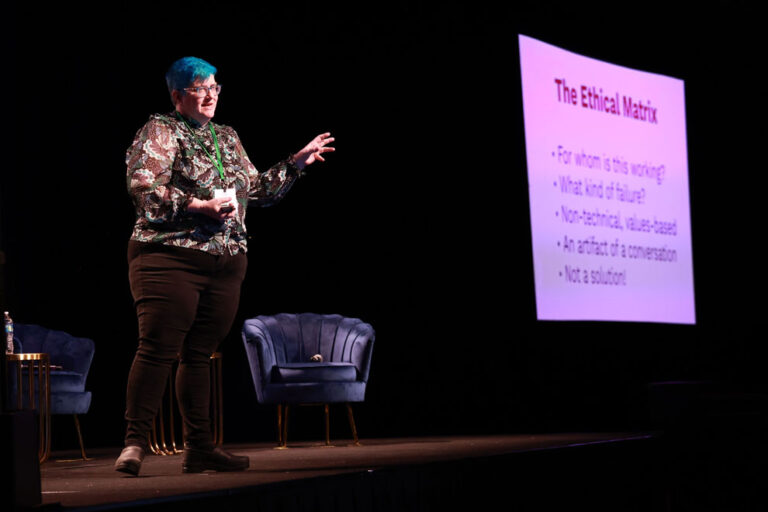
Cathy O’Neil — author of the books Weapons of Math Destruction and The Shame Machine, as well as founder of O’Neil Risk Consulting & Algorithmic Auditing (ORCAA) — was the featured speaker at the CAS 2022 Annual Meeting. O’Neil has been working with the Department of Insurance, Securities and Banking in the District of Columbia as they consider concerns about fairness in insurance pricing and underwriting.
The CAS Board of Directors in December 2020 approved a recommended CAS Approach to Race and Insurance Pricing, in which the organization committed to provide members and candidates education on disparate impact and research to develop methodologies that identify, measure and mitigate any impacts. Disparate impact refers to unintentional discrimination that can be introduced into decision making through such factors as unconscious bias, protocols rooted in and tainted by historical biases and biases inadvertently introduced into algorithms that drive hiring, operational and other decisions. In March of 2022, the CAS published its series of Research Papers on Race and Insurance Pricing that aim to fulfill the CAS education and research goals articulated in 2020.
O’Neil opened her remarks by sharing that she has wanted to speak to the CAS for a very long time, given all the profession does with algorithms. She developed a framework called “explainable fairness” that she employs as her practice audits algorithms across many industries and disciplines, and she believes it is applicable to actuarial practice within P&C insurance.
She defines an algorithm as the mathematical link between patterns from the past to predict the future. This requires data and one’s definition of success. In her book and auditing practice, she defines weapons of math destruction (WMDs) as meeting these criteria:
- Widespread — impacting a significant number of people
- Important — changing people’s lives in meaningful ways
- Mysterious and opaque — hard for those impacted to understand
- Destructive — adverse consequences to those impacted
She shared a few stories to help make her definition of WMDs more understandable. For example, Amazon developed a facial recognition algorithm that performed with 100% accuracy for the population on which it was calibrated — males with light skin tones. But when applied to other skins tones and gender, its accuracy dropped below 70% for darker females. Amazon’s algorithm had up to a 30% error rate, and the impacted populations would not know or understand why it was happening.
Fairness is not a mathematical question, but rather a decision criterion. Algorithms are not inherently racist — any racism comes entirely from how they are used.
When it comes to an algorithm audit to determine if it is a WMD, the auditor needs to answer several open-ended questions:
- What is the exact context?
- How do we define fair?
- How do we set thresholds?
She explained that defining fairness must be situational to the context of how algorithms are used.
“Fairness is not a mathematical question, but rather a decision criterion,” O’Neil said. “Algorithms are not inherently racist — any racism comes entirely from how they are used.” She used the metaphor that we need monitors around algorithms like a pilot needs dials in their cockpit to safely fly complex airplanes.
When ORCAA is invited to conduct an algorithmic audit, she develops the two-by-two “ethical matrix.” The matrix helps to capture for whom this algorithm works or for whom it fails. It also captures what kind of failure, or what failure looks like. This process is values-based and nontechnical. The output is an artifact of conversations, and it does not conclude with a solution.
Figure 1. Ethical Matrix
The ethical matrix captures all the stakeholders on the rows and the concerns on the columns. All stakeholders should be represented, and all concerns should be ranked. The auditor then populates and considers every cell in this exercise of practical ethics. Figure 1 is an example that O’Neil prepared based on a study about financial lending credit scores in the appendix of Equality of Opportunity in Supervised Learning by Hardt et al.
Hardt et al. were studying the relationship between the financial results of a hypothetical lender and different racial fairness standards. They applied five different standards of racial fairness and then modeled financial results.
O’Neil’s matrix concisely captured the three key stakeholders on the rows:
- the lending company.
- White customers.
- Black customers.
The columns then captured four key concerns in this study:
- company profitability.
- risk of false positives.
- risks of false negatives.
- credit report data quality.
By completing each cell in the matrix, the auditor can begin to form a perspective on the fairness of how the credit-lending algorithm is being applied to their customers.
As shown in Figure 2, profits are maximized when the lowest standard of fairness is applied and decreases as stronger standards of fairness are applied.
Figure 2
This outcome limits individual companies’ willingness to implement fairer algorithms in a competitive market. If society wants a fairness standard to be applied, it will need to be mandated and enforced consistently across the market to maintain a level playing field. O’Neil reinforced her message that fairness is a societal decision and not a mathematical construct in and of itself.
O’Neil then transitioned to a primer on ORCAA’s new explainable fairness framework. Her hypothesis is that when people ask to have an algorithm explained to them, they are not asking for an explanation of the math, machine learning or AI. Rather, they want to understand how and why the outcomes are fair. ORCAA’s audits focus on outcomes — how algorithms are used — and not how they are built.
“As an algorithmic auditor, I never care how an algorithm was built,” O’Neil said. “I don’t even care what the inputs of the algorithm were. I just care how it treats people.”
She focused on explainable fairness from the lens of a regulator focused on whether a law or regulation has been implemented fairly. Within the insurance industry, Colorado is leading the way with new fairness legislation, while other states are considering similar legislation or regulation. Key definitions are left to rule makers, so the industry knows how to run their businesses in compliance. In every situation, someone must define the outcome(s) of interest and the thresholds for fairness, which requires negotiation between regulators and the industry to make this all workable.
O’Neil shared two illustrative use cases for explainable fairness: student lending and disability insurance. In all cases, we must define the outcomes of interest for the situation and then measure outcomes for various interested classes. The negotiation comes into play when deciding which factors are considered “legitimate” and what “significant” means. These are not math problems, but rather legal and ethical decisions to be made in collaboration.
She closed by emphasizing the importance of values, monitoring and negotiation in the fair use of algorithms. To return to her cockpit metaphor, explainable fairness for algorithms requires a dashboard with many dials and metrics. The dashboard must be custom built for the specific algorithm application to assess fairness.
Dale Porfilio, FCAS, MAAA, is the chief insurance officer for the Insurance Information Institute.