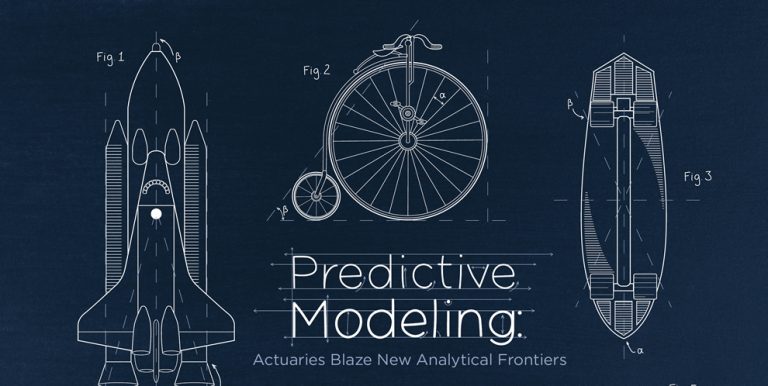
From solving insurance challenges through new applications for GLM to expanding into machine learning and other types of models — there is a lot of experimentation going on.
Even before credit scoring began revolutionizing personal auto insurance pricing, carriers have been on the hunt for more predictive modeling techniques and applications to outfox their competitors.
By relentlessly experimenting with combinations of analytical approaches and new data sources, actuaries are discovering insightful correlations for practical implementation. As generalized linear models (GLMs) are steadily expanding beyond pricing applications, other models promise new advantages.
Taking a closer look into the latest in predictive modeling requires examination of applications, types of models gaining acceptance, modeling approaches and other trends.
With all of these applications, there is plenty of experimenting taking place. When successful, experimentation leads to emerging innovation, which gains acceptance and gradually becomes common practice. Potential applications for predictive modeling are also exciting.
Many models that have been applied in other industries are new approaches in the insurance industry. The growing actuarial interest in predictive modeling represents one important trend, sources observed. Actuaries have become much more interested in predictive modeling than they were five or 10 years ago, said Christopher Monsour, vice president of analytics at CNA. “I remember being told 12 years ago that no one was going to pay for a more accurate reserve estimate,” he added. “Times have changed.”
Serhat Guven, Willis Towers Watson’s P&C sales practice leader for the Americas, offers some possible reasons why predictive modeling is growing. As actuaries become more educated, they are finding more modeling options beyond GLMs. These include the R programming language, vendor software and greater data access.
As predictive modeling evolves, nomenclature also matures. Currently, models are difficult to categorize. The same model can have different names. Terms such as “advanced analytics” or “more sophisticated models” can have different meanings. For this article, these terms refer to models beyond basic GLMs or decision trees such as unsupervised models and machine learning.
GLMs and Decision Trees
Employing GLMs for pricing is the only predictive modeling application that has truly become common practice so far. For application and deployment, actuaries are using basic GLMs in most cases for additional applications, Guven said.
According to the Willis Towers Watson’s 2015 Predictive Modeling and Big Data Survey, released in February 2016, 88 percent of those surveyed said GLM is their primary modeling methodology. As for approaches they plan to use in the next two years, 19 percent of respondents plan to use GLMs for the first time or in other operational areas.
A model advances according to insurer interest in its application. For instance, mobilizing GLMs to project claim settlement amounts is saving insurers “real dollars,” said Roosevelt C. Mosley, principal and consulting actuary for Pinnacle Actuarial Resources, so its adoption is progressing toward becoming a common practice.
Meanwhile, using GLMs for claims triage, which assigns claims to the appropriate examiners according to predictable severity, has been around for a while but adoption has been gradual, said Louise Francis, founder of Francis Analytics and Actuarial Data Mining, Inc.
According to the Willis Towers Watson’s 2015 Predictive Modeling and Big Data Survey, released in February 2016, 88 percent of those surveyed said GLM is their primary modeling methodology.
Using GLMs to operationalize the claims triage model is a new development, Guven said. Applying stochastic loss reserving with GLMs for loss reserving is also emerging.
Actuaries are also working with sophisticated kinds of GLMs — such as double or hierarchical GLMs and non-parametric GLMs, observed Peggy Brinkmann, a principal and consulting actuary for Milliman, Inc. “We are not at the end of the line with GLMs,” she added.
Brinkmann noted that decision trees are gaining greater use by actuaries who are not necessarily predictive modelers. “Decision trees have gone mainstream. There are more uses for them than just making loss cost models,” Brinkman said. Decision trees are also effective for finding patterns, anomalies or errors for exploring data. Their growing popularity is reflected in the Towers Watson Survey, which reports that 31 percent of respondents are using decision trees, with another 26 percent planning to use them in the next two years.
In the claims arena, some insurers are finding decision trees helpful for automating the detection of claim subrogation potential to make it more objective, Francis said. “They take the insurer’s history and look at what kinds of claims are subrogated and the outcome and use it to create a system to flag subrogation potential,” she explained.
Decision trees have been assigning claims to the appropriate examiners according to claims severity for quite a while, Francis said. They are being applied in personal auto, workers’ compensation and business interruption coverage, Guven said, and they also detect potentially fraudulent claims.
According to Mosley, decision trees also are effective for optimizing report ordering, which is commonly used for underwriting. “These models use the characteristics of the policy to predict whether there will be actionable information in the external information obtained,” he added. This helps determine the benefits of ordering reports such as property inspections and motor vehicle reports to reevaluate policyholders.
Advancing Analytics
GLMs are good for loss distribution analysis of insurance products, but do not necessarily work for all types of questions, said Mary Jo Kannon, an adjunct instructor at St. Joseph’s University. As a result, “actuaries are now working in nontraditional areas to use predictive analytics to solve different problems,” she added.
Applying advanced analytics is slowly starting to grow, Guven said. “There are more and more case studies around product teams deploying sophisticated analytics solutions beyond GLMs,” he added.
Only three percent of respondents in the Towers Watson survey said they were currently using “other” methods such as vendor products and non-GLM multivariate methods, though greater use is expected. Actuaries “are always testing for potential of other types of models,” Kannon said. However, Kannon has yet to observe a lot of models built out that are not GLM being used for actuarial projections.
Advanced analytics, which range from unsupervised models to machine learning, offer several benefits. In general, Guven said, they can provide better accuracy and are more difficult for competitors to copy. Formula-driven algorithms, rather than table-driven ones, can be easier to program in downstream systems.
As business applications expand to marketing, claims and other decision models, GLMs may not always be the go-to choice. “There’s no particular reason to stay with GLMs as opposed to other types of models. People often don’t — even for pricing models,” said Monsour. “There’s no compelling reason to use GLMs if you model frequency and severity separately,” he added.
In pricing, there is definitely more experimentation with advanced analytics taking place, Guven said. This is especially true for personal auto and major insurance lines including workers’ compensation, commercial auto and businessowners’ policies (BOP).
Applying sophisticated models for segmenting markets to improve marketing is also gaining popularity, Mosley said. “This is a fairly hot topic and gaining more momentum,” he added.
Such models are being used for looking at the expected likelihood of writing and retaining a client, competitive position and expected market profitability. In the past, Mosley explained, marketing was more judgment-driven, but as more data becomes available, market segmentation is improving.
As business applications expand to marketing, claims and other decision models, GLMs may not always be the go-to choice.
Unsupervised models such as clustering analysis, association discovery, sequence discovery and market basket analysis are also emerging. “There is a movement toward applying unsupervised models that employ clustering techniques to understand the nature of the data and how the data aligns,” Mosley said. Guven is seeing greater use of unsupervised models for factor identification and feature selection.
Since unsupervised models do not require a target variable, they are effective for identifying suspicious claim indicators or outliers. Mosley explained that they also are useful for detecting fraud potential, though this approach is not yet widely used.
Learning via Machine
Machine learning, another burgeoning insurance industry predictive model, concurrently applies several different modeling techniques to discover the best answer, Guven explained. This does a better job of identifying data signals compared to GLM and decision trees. There are hundreds of machine-learning models, including neural networks, gradient-boosting methods (GBMs), genetic algorithms and random forests.
he most frequently cited predictive model by sources is the GBM [gradient-boosting method], which is also called stochastic gradient boosting. A GBM “selects” the right approach by using hold out samples as the primary means of testing to realize the best of different approaches to drop the worst, Guven said.
“What is new is [that] actuaries are trying more techniques beyond GLM to improve the lift of the claims model,” Guven said, “and a small percentage are attempting to optimize machine learning for putting an algorithm out there that can learn on a daily basis as new data comes in.”
Willis Towers Watson’s survey reports that 12 percent of respondents are using machine-learning techniques with another 43 percent planning to do so in the next two years.
The lift is usually framed in a business context such as closing claims more quickly, improving satisfaction surveys and reducing claims costs, Guven said. Models are supposed to improve the business and the lift in the model is a measure (both prospective and retroactive) of that improvement, he explained.
The most frequently cited predictive model by sources is the GBM, which is also called stochastic gradient boosting. A GBM “selects” the right approach by using hold out samples as the primary means of testing to realize the best of different approaches to drop the worst, Guven said. “The key advantage is it produces more accurate predictions,” he added.
Since GBMs can process through different layers of potential variables, Guven said, they help actuaries identify a more homogenous risk segment at a deeper level of sophistication than GLMs.
Brinkmann cites several uses for GBMs. “If you are starting with a blank sheet of paper (for) a new model, there are a lot of new variables to evaluate and GBMs are useful … because they do not have all the assumptions and preprocessing as a GLM does,” Brinkmann said. GBMs also can identify new variables or develop new scores that can be used as variables, she added.
Artificial neural networks, generally called neural networks, are another form of machine learning that is enjoying greater experimentation. Neural networks are nonlinear models that mimic how the brain works to estimate or approximate1 functions that can depend on a large number of input variables to answer a question.
They step from layer to layer through a series of models applied from within the different layers, Mosley said, and are more powerful and flexible than other types of models.
“The ultimate goal is the network helps you, theoretically, more accurately predict the outputs based on the inputs you have,” Mosley said. Some neural networks have been used for pricing to an extent, Mosley said, and are also being used for claims triage because of their more flexible structure. These applications, along with retention and conversion analysis, remain in the experimentation phase with limited adoption, he added.
With so many exciting developments in predictive modeling, a model’s complexity does not assure its effectiveness for an application. Guven noted that choosing the correct model to use depends on many factors (see sidebar).
Disadvantages
While approaches beyond GLMs and decision trees are alluring, they also come with downsides, sources said. It takes time and experience to use the proper model that will help make a signal discernible and valuable, said Stephen J. Mildenhall, a professor at St. Johns University’s risk management and insurance department. “You are fine-tuning down to quite a granular level,” he said, which requires experience to know the difference between an actual signal and a spurious one.
Although advanced analytics are powerful for detecting data noise for the particular segment being modeled, they are not flexible to changes, Guven said. “Formula-driven approaches can be awkward to use when making minor tweaks compared to tabular-driven GLM approaches,” he added.
Guven remarked that machine-learning algorithms are not introspective, so they do not indicate why they are generating a bad risk. “The more sophisticated the model, the greater the complexities of the resulting segmentation,” Guven said.
For personal automobile insurance, as an example, segmentations of greater degrees make it more difficult to determine what will happen to premium when a customer moves from one segmentation group to another as his or her customer characteristics change. “If you want to stay in your market footprint, (machine learning) can be a great tool,” Guven said. “But if you want to grow into new market footprints, machine learning struggles,” he observed.
Actuaries have access to more data, more sophisticated techniques and a better infrastructure, but it is essential to communicate a model’s purpose and benefits to internal and external stakeholders, Guven said. This is difficult because greater sophistication also makes the reasons behind the results less transparent and harder to explain. “Product teams need to weigh the benefit of the added lift versus the need for transparency,” Guven said.
When models are difficult to explain to information technology professionals, implementation can be difficult, Francis said. However, visualization techniques can help explain more complex models, she added.
Conclusion
Thanks to greater data sources, technological improvements and experimentation with modeling techniques and applications, actuaries are venturing into new frontiers of innovation to boost predictive accuracy.
GLM and decision tree applications continue to expand and gain popularity. Advanced analytics promise greater levels of accuracy, yet their complexity is challenging to master and to communicate to users internally and externally.
While predictive modeling experimentation shows great promise, there are other considerations that will affect which strategies will move forward and stand the test of time. The third installment of Actuarial Review’s look into the latest in predictive modeling will cover topics including regulation, data ethics and the future data-and-analytics-driven insurer.
Annmarie Geddes Baribeau has been covering actuarial topics for more than 25 years. Her blog can be found at http://insurancecommunicators.com.
1 https://en.wikipedia.org/wiki/Universal_approximation_theorem
So Many Techniques, So Little Time
With so many models to choose from, actuaries should consider several factors for selecting and working with predictive models.
When it comes to modeling, there are two ways to get a better prediction, explained Peggy Brinkmann, a principal and consulting actuary for Milliman, Inc. “You can try to use a different algorithm and/or add new variables to the model,” she said. “My experience is a good variable adds a ton of lift.”
Serhat Guven, Willis Towers Watson’s P&C sales practice leader for the Americas, recommends that modeling should not be viewed in isolation but rather through the process life cycle. “When you talk about modeling or product decisions, you have to think about it as the spectrum of how it impacts everything.” He stressed that “We cannot think of modeling in isolation of everything else.”
First there is the foundational component of gathering and collecting data, which must be in good quality and offer a depth of information, Guven said. “It’s not just about what you want to do but what data you have available that will shape what modeling techniques you take on,” said Jo Ann Kannon, an adjunct instructor at St. Joseph’s University. “If you have oodles and oodles of data,” Brinkmann said, “there are more options available to you.”
Model selection is next. This includes determining the model’s goal and understanding the business problem the model is to solve. The modeler’s preference and the software also play a role in the decision, said Louise Francis, founder of Francis Analytics and Actuarial Data Mining, Inc.
A simpler model is often preferred when modeling process is first being attempted, Francis said, because it is easier to explain to management and for deployment purposes. For some applications, Guven said, GLMs are still the best approach, as long as actuaries are using robust and quality data, because they are simpler and easier to explain than advanced models.
If management is most interested in accuracy, Francis explained, “They will go with an ensemble (or advanced) model, which requires substantial IT resources especially in the deployment phase.”
The third step is making a decision from the results. At this point, the question Guven asked is, “Should the product team trust the model wholeheartedly?” Pricing requires more of the expected impact from the model because if it is wrong, there is a lengthier process to change it, which includes regulatory approval. “Contrast this with operational claims models because [they] do not require external approval,” he added.
For advanced analytics, the product team needs to weigh the benefit of the added lift compared to the need for transparency, Guven said. Since advanced models are very technical and therefore less transparent, their use can depend greatly on how well the actuary communicates about them.
Finally, there needs to be consideration for how the model will be deployed. “A lot of resources are required,” Francis said. “Cost is involved in developing the model and there can be substantial additional cost when you deploy it,” Francis said.
“The real innovation requires change management,” Kannon concluded.
The cost of the delivery is not just in implementing a model but also should be considered in evolving the model, Guven said. “One of the responsibilities of the actuary is to be able to both prospectively assess and retroactively monitor how the improvement from the models outweigh the costs,” he added.