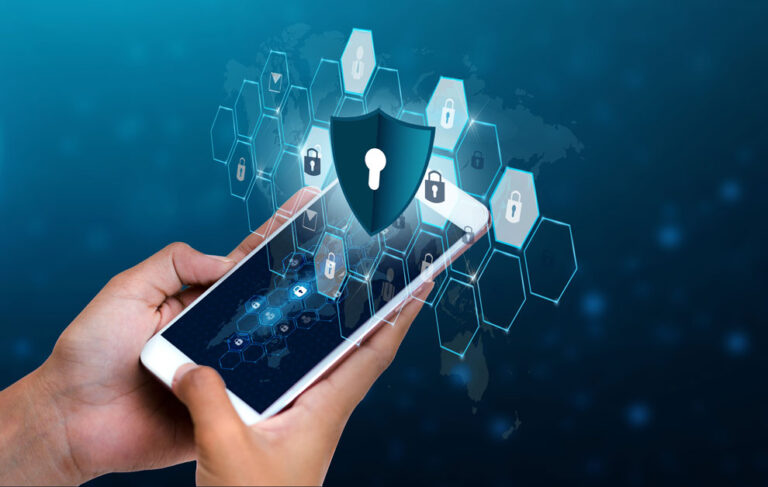
Most practicing actuaries make a living by using data to solve business problems, so Jennifer Golbeck was invited to be the keynote speaker at the virtual 2022 CAS Ratemaking, Product and Modeling Seminar to share a deeper message about the risks and opportunities of Artificial Intelligence (AI). In her remarks, Golbeck sought to warn us about the invasiveness of AI and the difficulty of avoiding bias when building predictive models. She shared many stories from her own research and from other industries to reinforce her key messages.
Golbeck is a computer scientist, director of the Social Intelligence Lab and a professor in the College of Information Studies at the University of Maryland, College Park. Her research focuses on analyzing and computing with social media and creating usable privacy and security systems. She began her research in AI and social media while a Ph.D. student with a lab of undergraduate students, and she has continued to expand upon her work with social media.
Golbeck shared several examples about Facebook, given its pervasive data collection. A key underlying principle in AI studies is homophily — our tendency to connect with people like ourselves. Leveraging these connections, Facebook creates a profile for everyone it can identify in its environment, including those who have never created their own Facebook profiles. They are ready to greet you as soon as you consider becoming a member!
Cambridge University did deep AI modeling of Facebook’s user “likes” (all in the public domain) to predict demographic and personality traits of users, including IQ. They found four leading likes that predict a high IQ — science, thunderstorms, The Colbert Report and curly fries. Golbeck made clear that it is not necessary to understand why something may be predictive, since we are merely studying correlations to make predictions.
Target built a model based on purchasing history to predict which customers may be pregnant. Its model identified the three strongest predictors for pregnancy — excess lotion, handbags (large enough to double as a diaper bag) and brightly colored rugs. Again, it’s not essential to understand why anything is predictive. Target used this model to mail coupons for maternity and baby needs to its customers, including a 15-year-old young woman whose parents did not yet know she was pregnant.
Golbeck then emphasized that while AI has a veneer of objectivity, AI models can easily be used for adverse social purposes if we do not have sufficient regulations or controls in place. For example, she and her lab studied Alcoholics Anonymous participants to predict who would be successful at staying sober for 90 days after attending their first meeting. Golbeck’s lab was able to achieve an 85% prediction rate, which is a great result for statisticians but leaves ample room for false results. If this model were used to decide sentencing following DUI convictions, the 15% error rate could allow many lives to be adversely impacted.
For those who may naively trust that all companies will respect our privacy settings, Golbeck transitioned to examples of the data collection happening all around us:
- The Spanish football league, La Liga, turning on smartphone microphones of La Liga app users and listening to determine whether users were watching La Liga matches in bars that had not paid for broadcast rights.
- Facebook performing photo matching and phone movement/orientation matching to suggest new connections in their “people you may know” feature.
- Apps on Golbeck’s phone sending local ads by accessing Wi-Fi hotspots near her device, even though she was using VPN through a different state to mask her location.
After clearly conveying her message that we have ample reasons to be concerned with how much data is collected on us and how it is used, Golbeck shared her advice for how to use AI effectively and responsibly. This included a book recommendation for Weapons of Math Destruction: How Big Data Increases Inequality and Threatens Democracy by Cathy O’Neil (see the AR November-December 2016 book review). In discussing the book, Golbeck focused on three key issues:
- Transparency — We should be prepared to disclose our algorithms and how we are using them.
- Consent — People want the right to give permission (or not) for how their data is used. This requires a company to exhibit a higher standard of care than merely being compliant with the terms and conditions (which we all accept without reading). She advocated for the U.S. adopting Europe’s stronger privacy and consent rules for the use of personal data.
- Bias — Algorithms are not trustworthy for some applications. While 75%-90% prediction accuracy is great for science, we need to study the error rates for potential bias.
She shared two powerful examples of highly predictive AI applications with significant bias. The first was a Silicon Valley firm that built an AI model to assist with its recruitment of engineers. Many tech firms have a poor record of hiring, developing, promoting and retaining women engineers. This model was built using the firm’s own experience, and the results ended up reinforcing its prior sexist behaviors.
The second example involved a health care algorithm built using hospital records of patients with comorbidities. The algorithm indicated who should be referred to an expense mitigation program. The algorithm was trained to predict future health care costs (which it successfully did), but it was biased by race and referred too few Black patients because Black patients tend to have lower health care costs than whites. Once the bias was identified, the model was retrained to incorporate prediction of future health outcomes in addition to cost, which better balanced the referral rates between races. Two graphs capture the model outcomes before (Figure 1) and after (Figure 2) retraining for the racial bias.
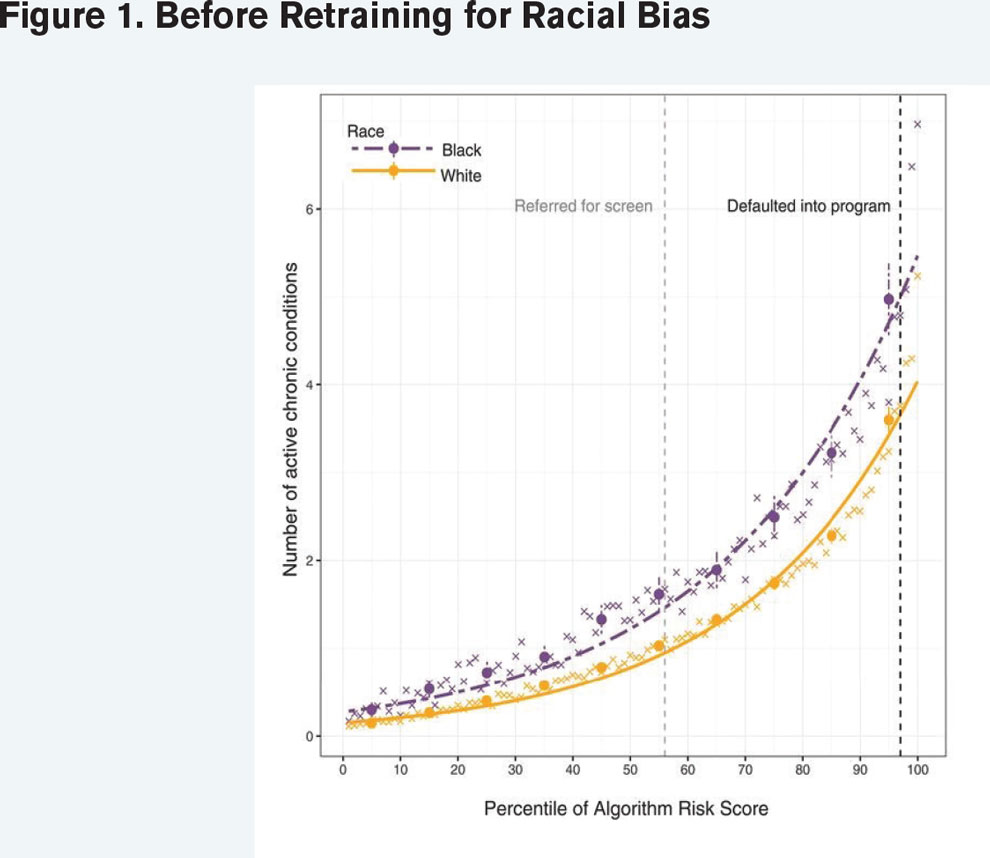
Populations.” Science 366, no. 6464 (2019): 447–53. https://doi.org/10.1126/science.aax2342.
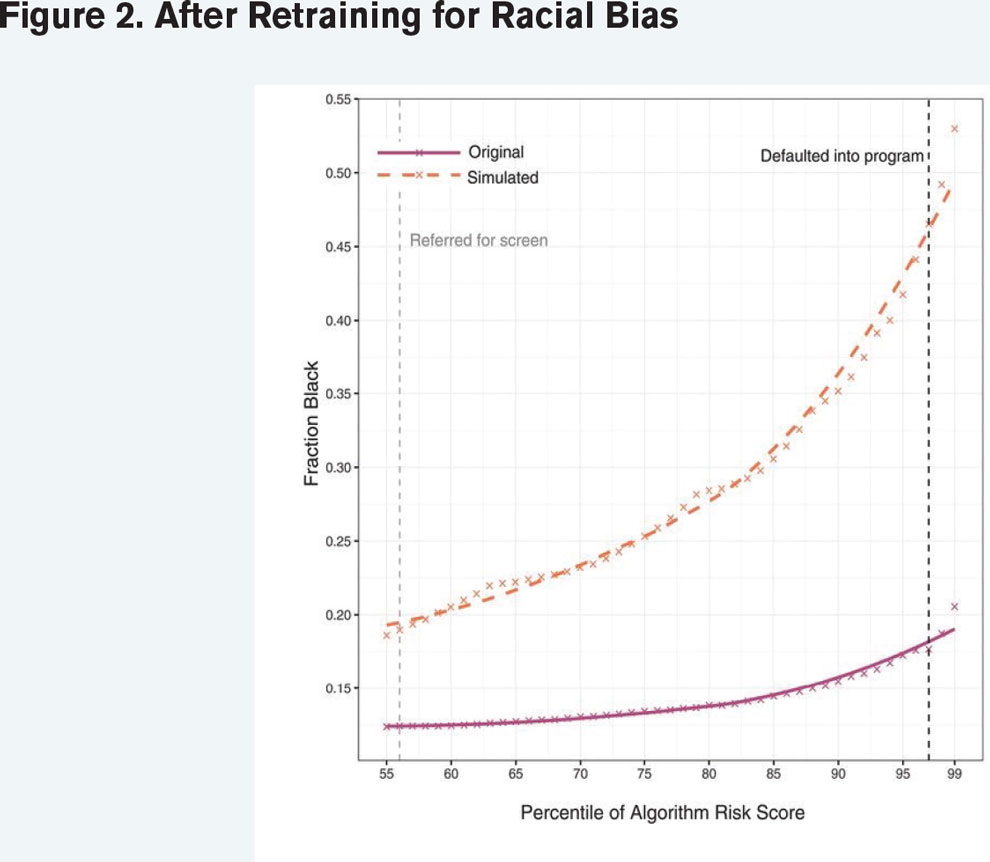
Golbeck concluded her remarks by summarizing her main points, then opened the virtual floor for Q&A. She wanted her audiences to be aware of the invasiveness of AI prediction technology and data collection, and what this means for our personal privacy. More specifically, she challenged the actuarial profession to test for bias in the results of our predictive models to ensure that we are providing high-quality and socially conscious work for the insurance industry.
Dale Porfilio, FCAS, MAAA, is chief insurance officer for the Insurance Information Institute.