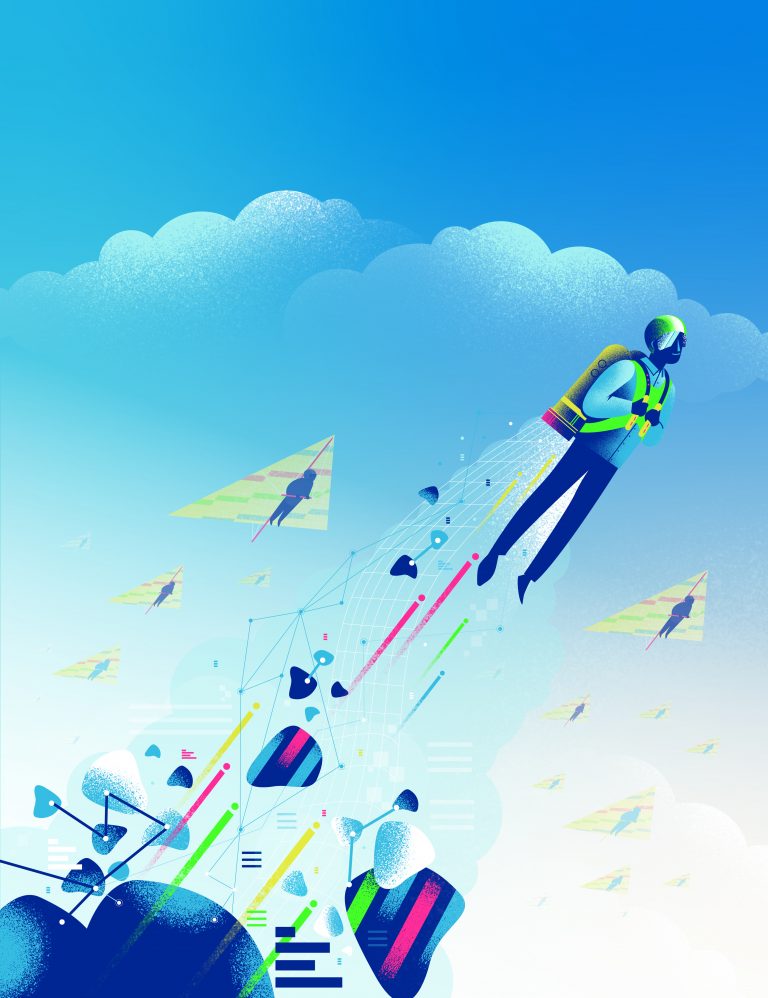
Thanks to data analytics, reserving will never be the same.
Oh, reserving! It is the bread and butter of actuarial work. Required by state statutes to have an actuarial opinion, reasonable reserving estimates on insurer balance sheets essentially represent a survival-critical assurance of solvency.
At insurance companies, reserving actuaries are “people of power,” said Christopher Monsour, FCAS, data science practice regional vice president for the Americas at DataRobot. “They are the scorekeepers — the judges.”
For nearly a century, actuaries have based reserving calculations on claim cost estimates first made by claims adjusters, then aggregated through tried-and-true triangles to produce the incurred but not reported (IBNR) estimates.
But that is changing.
Thanks to technological advancements supporting more sophisticated models, some actuaries are turning the reserving process on its head. Rather than start with claims data aggregated through triangles, pioneering actuaries are beginning with unaggregated claims data. They are also applying modern models far more powerful than traditional generalized linear models at the claim, coverage and policy level.
Coming up with individual ultimate reserves first at the claim and coverage level, and then adding in pure IBNR estimated at the policy level, marks a “fundamental change in the starting point” in reserving, said Ken Williams, FCAS, staff actuary for the Casualty Actuarial Society (CAS).
“You want to analyze and then summarize, rather than summarize and then analyze,” Monsour explains, thus making it much faster to respond to new questions requiring new summarizations.
Still considered an experimental practice, more insurers are implementing this new approach to reserving.
What’s more, the new methods can improve operational practices for actuaries, claims professionals and underwriters through a feedback loop to benefit insurers’ organizational goals, said Zack Martin, FCAS, FSA, CSPA, vice president of actuarial, risk and compliance services for Frankenmuth Insurance. (For purposes of this article, models that use current technological advancement are called “advanced analytics.”)
Monsour predicts the practice will become mainstream in the next five years. “Executives will want answers faster and faster and this is how actuaries will fill the need,” he said. Despite the promising multifold benefits of applying advanced analytics to unaggregated individual claims data, there are several barriers to overcome in an industry sometimes set in its ways.
Despite the promising multifold benefits of applying advanced analytics to unaggregated individual claims data, there are several barriers to overcome in an industry sometimes set in its ways.
Reserving Re-Think
Fundamentally, reserving principles and practices are rooted in a rudimentary pre-computer calculation process from the 1930s that was later modernized in a 1972 Proceedings paper, said Chris Nyce, FCAS, an actuarial services principal for KPMG. Calculations automated with reserving software are primarily based on formulas from the 1970s applied to data aggregated through triangles, explained Nyce, who co-authored “Loss Reserving in the Future: Innovation in a Rapidly Changing World.”
The approach made sense in the past, said Brian Fannin, ACAS, CSPA, CAS research actuary, because triangles were available and easy to use. Although triangles have generally been sufficient for evaluating reserve estimates, some may find them limiting. “The triangle data structure is rooted in financial reporting, not statistical analysis,” Fannin observed. Detail is aggregated away to create triangles, he said, eliminating granular information that actuaries would like to see in more detail to realize the range of possible outcomes.
Even without advanced analytics, there are benefits to merely exploring claim data, said Kevin Kuo, chief technology officer at Hal9, a new firm specializing in artificial intelligence. “Just the exercise of preparing to do individual claims reserving forces you to look at the data for more perspective,” he added. “You might get the IT folks to gather more data that will allow you to do more.”
To know if a model is appropriate, Fannin recommends techniques such as cross-validation, which splits data into training and test sets that can demonstrate the level of variability in model performance for future periods. However, Fannin noted that aggregate data does not have sufficient sample points to make cross-validation meaningful, another argument favoring more granular data.
Other technology, including automation and visualization, can be applied hand-in-hand with advanced analytics for the most beneficial results. Automation supports advanced analytics by handling repetitive tasks, Nyce said, such as data manipulation. “If you can define the process, you can develop a macro to do it,” he added.
Although data presented in triangles is limited, squared triangles can serve as tools for communicating projections to management and investors, Monsour offered. Additionally, machine learning visualization tools have greatly improved in recent years, and “good visualization can drill down to show which claims features drive the model, and thus provide strategic insights,” he added.
Within a choice of drop-down menus using visualization tools, Nyce said, “You can take a look at the profitability of a book any way you want,” by aggregate, detailed or financial reporting views of the output. Without visualization and automation, seeing the same results requires a team effort to process and display the results. “You would need to have triangles broken out for segments you wanted to evaluate, but now it is easily available in the output of advanced analytics,” he said.
Perhaps most importantly, visualization can help demystify the “black box,” which is essential to moving advanced analytics for reserving forward, Monsour said.
Bountiful Benefits
Modeling individual claims data offers multifold benefits that not only promise quicker and more detailed estimates but other advantages that can ripple through an insurance company’s operations to boost efficiency in claims, pricing and underwriting. “Generally, modeling individual claims will mean a more complex model with more moving parts than when you model at an aggregate level,” Nyce said.
Running individual claims data through advanced analytics quickens the reserving process by eliminating the need to use last year’s reserves and repeat the same calculations for this year to redo the reserve analysis, Nyce said. Actuaries can now plug the detailed data at any valuation into the existing model using current parameters and get a known claim and pure IBNR result.
“That is where the momentum comes in!” he said. “Once you have the model, you have a set of parameters allowing you to plug the most recent data in and get an answer. You could do this a week before close to get an advanced view of the closing results, for example,” he added.
There are other advantages to applying advanced analytics to unaggregated individual claims data that can enhance decision-making across different functional areas of an insurance company.
Further, the reserving process can be more fully automated for a second opinion or finding complex patterns using individual claims data. Considering credibility in interpreting results, “Now an actuary can provide an IBNR estimate by choice of claim, coverage level, state, ZIP code groupings and class of business,” Nyce said. “By giving actuaries access to more complete data and additional variables, actuaries can anticipate a claim’s cost potential, flagging severe claims earlier,” he added.
Actuaries can also see, for example, why aggregated reported losses develop differently over time. In one case, Nyce said, underwriters were writing a significant class at a 150% loss ratio, and advanced analytics revealed the primary culprit.
When claims are better understood, reserving estimates are as well. Because models are more sophisticated up front, Monsour explained, additional work is not needed to drill down into individual claim level projections: Those details are also provided by the model.
There are other advantages to applying advanced analytics to unaggregated individual claims data that can enhance decision-making across different functional areas of an insurance company. Martin said that the ability to utilize all the data opens many possibilities.
“Just providing granularity to what is driving losses can be used for strategic decisions for underwriting and pricing,” he said, including more refined insights into what is driving profitability. When reserving actuaries are developing estimates based on current claims activity that is more informative to pricing actuaries, the feedback loop of shared data between reserving and pricing actuaries becomes more robust, he explained. Underwriters can benefit as well.
The approach also improves other operational efficiencies. Detecting elements that can predict potentially severe claims supports both claims professionals and actuaries.
When claims examiners enter key terms or phrases in the text of a claims file, collecting and analyzing that data can help triage claims, resulting in more effective claims management. Certain terms can also assist actuaries to arrive at more accurate claims estimates.
Flagging the name of an opioid prescription in a claims file, for example, can signal potential complexities and difficulties in that claim, Nyce said. Of course, claims models and profitability models have been around for some time, but now one model can have multiple uses and be one version of the truth, he added.
Williams said that insurers might also make changes to achieve faster claim closure, which reduces the number of open claims requiring reserve estimates and thus the overall reserve estimate. “In the past, there were not great ways to account for that in the reserving process, but reserving at the detailed level picks it up automatically,” Williams said.
Using granular claim attributes also contributes to accurately projecting losses. The models allow actuaries to get information for many different ages and types of claims at once because they are more flexible, said Monsour. He began experimenting with generalized linear models for reserving about 15 years ago but found the machine-learning approach to be more flexible. Importantly, advanced analytics makes it easier to tell how much development is from open or closed claims, or from large claims or small claims, Monsour said. This can help actuaries determine how the reserves should be split between primary and excess insurers or between the direct insurer and excess reinsurer.
Seeing trends and patterns up front also allows actuaries to analyze claims information in ways not before possible. “Being able to forecast payments period by period until the claim is closed helps with cash flow management,” said Kuo, who wrote the CAS Research Paper, “Individual Claims Forecasting with Bayesian Mixture Density Networks.”
Applying advanced analytics to unaggregated individual claims data supports detection of claim anomalies, new variables that become important, or complex constellations of patterns that can alert actuaries to the potential cost and duration of a claim, Nyce said.
“Locating new variables and impacts can also help actuaries better predict scenarios that may take claims longer to settle, such as the likelihood of a claim going to litigation, or help claims personnel address the reason why claimants hire lawyers,” Williams said.
Also important, machine learning allows actuaries to make changes or adjustments, Williams said. Traditional claim reserving methods rely on assumptions based on how claims were settled in the past even though these patterns can change, he said.
By analyzing claims data more thoroughly through advanced analytics, claims adjusters can make better reserve estimates before actuaries begin their calculations, Williams observed. Rather than waiting for the next reserving cycle, actuaries can also track claims data to adjust reserves quickly due to changes such as inflationary pressure or the impact of COVID-19.
Barriers to Adoption
Although the potential benefits of applying advanced analytics to individual unaggregated claims sound promising, there are several hurdles to overcome before the approach realizes widespread adoption.
For starters, the methods are still experimental. Martin said that there is “not a set of consistent approaches to how a practitioner would go about developing a reserve estimate using the granular claims approaches.”
Rather than waiting for the next reserving cycle, actuaries can also track claims data to adjust reserves quickly due to changes such as inflationary pressure or the impact of COVID-19.
“Most companies do not have the resources to do it right,” said Kuo. Applying advanced analytics to individual claims reserving requires access to data, computing infrastructure and an actuary with data science expertise who can execute building the model, he explained. Assembling this combination is currently difficult to achieve.
Additionally, there is a lack of publicly available data concerning exposures, or policy-level information, to train models for pure IBNR, Kuo said. So, research progress has been limited for IBNR projections at the individual claims level. In general, data is probably not as much a concern for big carriers as it is for medium and small insurers. “The problem for small insurers is…they might not have enough data to build even a model on only reported claims,” Kuo explained. For prototyping algorithms, he recommended an open-source simulator for individual claims data at https://blog.kasa.ai/posts/simulation-machine/.
Williams offers another obstacle to implementation. Since more accurate reserving is not seen as providing a competitive advantage like pricing, it is more challenging to convince practicing actuaries and insurers to adopt it. He said that the challenge for reserving actuaries is to convince company leaders and clients of the benefits of better reserve analytics for not only reserving but potentially enterprise risk management, pricing and claims analytics.
Insurance adapts more slowly than most industries, Nyce said. Realizing the advantages of individual claim reserving, however, requires insurers to be willing to re-think 50 years of actuarial reserve practices. “However, the advantages of understanding profitability at any cut of the data, you can imagine, is a huge competitive edge,” he offered.
“The biggest challenge,” Monsour said, “is how to provide the appropriate diagnostics around the model so they are not a ‘black box.’” Nyce said that the auditing process, which is embedded in regulations, will also need to adapt.
From a practical point of view, it is easier to “stay with the triangles,” Nyce said, than use new granular data approaches. That’s because it takes creativity to understand more complex models, creating the need to audit more detailed data. “Especially when used for reserving,” he added, “there has to be acceptance and trust of the new methods by regulators and auditors.”
Conclusion
When advanced analytics were introduced in personal lines pricing more than 20 years ago, it took more than a decade to become a best practice. Fortunately for reserving actuaries willing to push the barriers of convention, the evolution of sophisticated modeling for pricing offers a pathway of lessons learned that could advance new reserving approaches more quickly.
Insurers have also become more open to the potential that advanced analytics offers throughout their organizations. Just as insurers rely on actuaries to assure solvency, the industry’s original data scientists should lead the way for advancing new approaches to reserving.
That means reserving actuaries must challenge themselves to look beyond triangles to improve reserving practices. Fortunately, actuaries now have the CAS Institute, which offers specialty credentials in quantitative practice areas such as predictive analytics and data science — this should ease and quicken the transition from the traditional reserve methods to modern approaches. ●
Annmarie Geddes Baribeau has been covering insurance and actuarial topics.