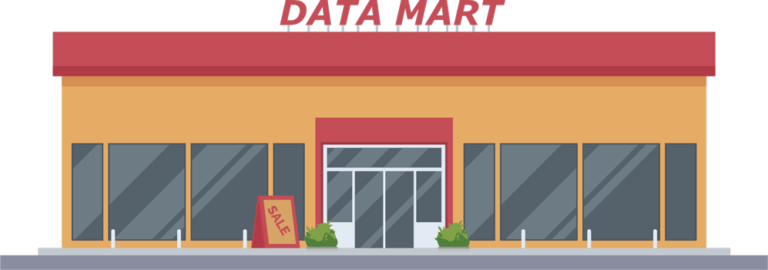
Do you like your data organic?
Imagine you are hosting some dignitaries at your home for a delicious meal tonight. Feel free to choose whichever dignitaries you wish to imagine hosting, but for the sake of argument, let’s assume we are wining and dining with CEO Victor Carter-Bey, CAS President Frank Chang and CAS Board Chair Roosevelt Mosley. Where will we be shopping for our ingredients — the local ShopRite, Whole Foods or perhaps the nearest farmers market? Suppose besides dinner, we are also serving these gentlemen some freshly baked actuarial analysis for dessert. Where are we shopping for the analysis ingredients? In either scenario, the final taste left behind may depend in part on our shopping decisions.
Verdant pastures, unpasteurized
To provide our guests with the most organic experience, we may travel to a local farm, pick some fruits and vegetables, milk a few cows and perhaps herd some livestock into our trunk. When we manage to get these items home, it will likely be a grisly and time-consuming endeavor to make them edible for our guests — but there will be little doubt as to the items’ freshness or quality.
Similar to our farm, a data lake contains fresh, uncontaminated ingredients such as web server logs, clickstreams or telemetric data, in native formats such as JSON or XML. For example, if a prospective policyholder obtains a quote over an insurer’s website, all the information accumulated during that journey — such as entries and selections, clicks and uploads, as well as any information permissibly retrieved via third-party web services — may be stored as a single semi-structured object and sent swimming in the lake. We may need to wield some tools that we are less familiar with, such as Python, to locate these items and render them suitable for analysis, but at least we know the exact path they took from farm to table. As we become more familiar with the carving tools, we may become more adept with them, or we may simply decide to befriend other skilled professionals such as butchers or web developers who can help us prepare our food and data from farm/lake to table in the future.
Similar to our farm, a data lake contains fresh, uncontaminated ingredients such as web server logs, clickstreams or telemetric data, in native formats such as JSON or XML.
Unfortunately, the time and effort required to execute effectively here likely renders the lake impractical for the present predicament.
Aisles upon aisles, shelves upon shelves
Rather than reaping and butchering our own ingredients, we may instead pull over at Costco (assuming we are members). Here we will have economical access to virtually limitless supplies. We may need to buy in bulk — 50 filets and 100 potatoes to serve three guests — and take a hydraulic lift to reach the gravy. However, we can rest assured that everything we need will be neatly packaged, on a dustless shelf (row), on one side or another of a spacious, cryptically labeled aisle (column).
Similar to Costco, a data warehouse benefits from some artisan having already gone to the data lake and magically transformed JSON blobs and overgrown XML trees into endless supplies of majestic and well organized columns for us. Any quoting option or click sequence from the aforementioned user journey tagged in even one XML earns its very own column on every row of the warehouse. We all know columns (mostly empty or not) lend themselves very well to the types of longitudinal analysis used by actuaries, particularly in certain Microsoft Office applications that many of us excel at and use frequently.
If we have an elaborate vision and a fair chunk of the afternoon to devote to the cause, a data warehouse may provide amply for our guests — but it still requires prior knowledge to navigate, and it offers a lot of stuff we may not want or need.
Whatever fits on a microwavable tray
Running out of options, we may consider Walmart as a final resort. Here we will instantly feel welcome (thanks, greeter!), and the freezer section will not be far from the entrance. Within minutes, we will find four Hungry-Man Dinners that have proteins, potatoes and veggies all in the same package — not to mention dessert. (We mean dessert in both the traditional sense of a sweet treat following a meal, as well as in the figurative sense of the post-nosh actuarial analysis we promised the guests — assuming nutritional information broadly qualifies as actuarial.) Once home we can throw our four trays in the microwave, go set the table and hope for the best.
Similar to Walmart, a data mart benefits from someone having already rummaged through data lakes and warehouses and slapped together what they needed into a single “ready-to-eat” package. Do we need quoting, claims and customer satisfaction data for our analysis? So did some other person once! Their hard work will save us all the time we would have spent rummaging through lakes and warehouses. If their work does not exactly serve our purposes, we can sprinkle on some herbs (joins) and spices (transformations), serve our guests and write down the recipe for posterity. If the guests really like it (or pretend to), we might even open our very own data mart that regularly has the modified recipe in supply.
Data marts may not always yield five-star analyses, but sometimes a Hungry-Man dinner can be just what the doctor ordered.
Bringing everyone to the table
So where are we shopping for our dinner and analysis ingredients after all this? That will likely depend on several factors. An obvious one is the amount of time we have on our hands, with the less time we have, the further down the supply chain we’ll need to go. Another factor might be the tools at our ready disposal, whether those tools are open-source programming languages that can manipulate non-structured and semi-structured data (lakes) or the prior knowledge of how our data is organized (warehouses). The three guests I imagined do not strike me as fussy, but they probably expected more than microwaved Hungry-Man meals and recycled analyses — so our stakeholders’ expectations should also factor into our decisions. We may also consider whether we plan to serve this meal/analysis again and how others in our community might make productive use of the leftovers.
All else equal, I generally prefer to get my food from the farm and my data from the lake. In a practical sense, I often find myself in Walmarts or data marts buying Hungry-Man meals and analyzing glommed datasets. Either approach can be okay, but our default should not always be to pull over at the most nearby or expedient place we think has our supplies. As technology and our stakeholders’ needs evolve, so should we. Generative AI feasts on non-structured data and setting it loose in a mart would be like sending Gordon Ramsay to 7-Eleven. Moreover, even if we are meeting stakeholders’ expectations, we could potentially exceed them by availing ourselves of data that does not make its way downstream. Do we even know how much fell off the truck on its way to the data mart? If not, we should consider asking our nearest enterprise architect. Analyses need not be single sourced, so we can also mix and match, getting our vegetables from the farm, our proteins at Walmart, our premiums/losses at the data mart and our clickstreams from the data lake. We can also ask our dignitaries if they mind waiting a few weeks for a more worthy meal or analysis. The more satisfying experience we provide our guests, the more likely they will be to come back in the future.
Jim Weiss, FCAS, CSPA, is a vice president for Crum & Forster and is editor in chief for Actuarial Review.