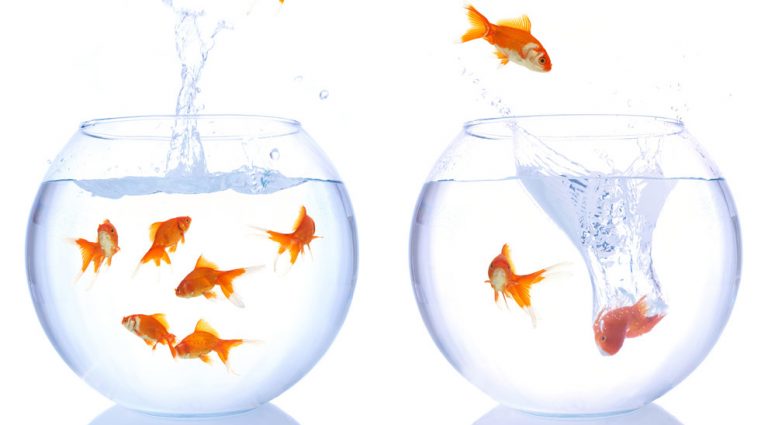
Editor’s note: This article is the second of a two-part series that highlights the careers of eight actuaries who are in the “other” category. Their roles and advice for success showcase what it takes to venture into unchartered territory. This article features four actuaries. Part One is published in the September/October 2017 issue of Actuarial Review.
CAS members working in capacities in the “other” category — those working beyond traditional roles at insurance companies or consulting firms — are solving diverse business problems through analytics.
They demonstrate the growing need for analytics capabilities, which will continue as big data and modeling reveal ways to solve business problems in all industries. Part Two of Actuarial Review’s series on the “others” features actuaries who are demonstrating analytical prowess in multiple ways.
These actuaries’ experiences illustrate the power of analytics in their careers. Like those covered in Part One, each actuary first worked in the insurance space before venturing off into new industries.
Deploying Predictive Analytics for Diverse Business Applications
Kevin Kuo, Software Engineer, RStudio, Inc.
After applying predictive analytics to enhance internet of things (IoT) related products at Honeywell International, Inc., Kevin Kuo recently joined RStudio, Inc. as a software engineer.
His goal: Enhance R’s open-source ecosystem to offer big data and deep learning capabilities to R users. “Initially I am focusing on sparklyr, which provides an R interface to Apache Spark, a distributed computing framework,” he says. “But I also plan to contribute to the R packages for TensorFlow and Keras, which are libraries for deep learning.” The idea is to enable professionals familiar with R to leverage the latest technologies and hardware without having to explore other programming languages, he explains.
Before joining RStudio in August 2017, he spent six months as a principal data scientist at Honeywell. There, he led the team that built predictive models using data from sensors and telematics devices as well as external topographical and climate data to improve predictive maintenance of automobile parts such as turbochargers. Instead of basing maintenance on just miles or time, the models consider other factors such as driving behavior and altitude changes. “A vehicle that goes up and down hills a lot in hot weather will have a different maintenance profile than one that just cruises on level roads in a mild climate,” he explains.
In addition to IoT applications, his team also built prescriptive models for the company’s accounts receivable departments to prioritize collections resources and to inform collections strategies. “The idea here was to use machine learning to identify which customers would be more likely to pay sooner if you reached out to them and how you should go about contacting them, for example via phone or in-person visits,” he explains.
While at Honeywell, he also had the opportunity to contribute to model deployment infrastructures. “After you have some R code to build a model,” he explains, “you have to turn the model into a service that can respond to real-time prediction requests from your end users.” Achieving this means scaling service to handle a high volume of requests and refreshing the model dynamically if needed. “This isn’t a topic data scientists are traditionally familiar with, but I’m seeing them adapt in order to be more effective in cross-functional teams,” he adds.
A Diverse Career Path
The predictive analytics expert could not have predicted that his career would lead to software engineering. Originally, Kuo pursued a career in finance. After earning a bachelor’s degree in applied mathematics, he started his career as an options trader at a market-making firm. Shortly after the financial crisis in 2009, he lost his position and played poker online for a while before returning to school to earn a master’s degree in applied mathematics.
“Don’t get too fixated on insurance knowledge … but leverage the fact that you have experience working with quantitative problems in a business context.” — Kevin Kuo
Kuo began his actuarial career in life insurance at KPMG, LLP. “I discovered that some of my financial mathematics knowledge was transferable to valuation of embedded options in variable annuities, so I decided to start there,” he says. However, he quickly pivoted to working on predictive modeling engagements due to project needs, which led him to transfer to the consulting firm’s P&C practice and to start taking CAS exams.
After a couple of years, he joined Citibank to lead the direct mail acquisitions strategy for the Home Depot credit card portfolio. “That was a marketing analytics type of gig,” he recalls, where he built customer response models and designed A/B testing experiments to evaluate different designs of mailers and offers. He returned to KPMG for a year and obtained his ACAS before joining Honeywell. “I just picked up more and more technical expertise around software engineering as I progressed in data science, mostly out of necessity,” he explains.
Kuo offers the following advice to actuaries considering opportunities in other industries: “Don’t get too fixated on insurance knowledge … but leverage the fact that you have experience working with quantitative problems in a business context,” he says. “If you’re interested in data science, you’ll also have to roll up your sleeves and start coding, especially if you’re in the early stages of your career.” While many people can talk the talk about bridging technology and the business, few can actually get solutions built, and those who can are the most in demand.
Optimizing Travel Insurance
Aaron Fezatte, Strategy Manager, Expedia, Inc.
Aaron Fezatte applies business intelligence to boost online sales of optional travel coverage.
Before joining Expedia three years ago, Fezatte was a senior actuarial analyst for Liberty Mutual Insurance Company, where he worked on pricing and analytics for both personal and commercial lines. At Expedia, he applies his skills from a different angle: as a member of the strategy team. His job is to secure coverage options for various exposures related to traveling. “My role,” he explains, “involves working with insurers to develop travel insurance products to meet the needs of our customers around the world.”
Several goals are involved with applying business analytics to the demand for travel insurance. One goal is that his team tries to ensure coverage is “as attractive and affordable as possible,” he explains. By slicing and dicing data, he can make recommendations to insurance companies and can encourage them to consider other approaches to coverage. “It is helpful to understand the insurance perspective and provide supporting data, ultimately enhancing the relationships with our partner insurance companies and giving better value to customers,” he says.
There are several variables that can affect offering the right product. Fezatte analyzes data ranging from type of travel, trip cost and destination to length of stay and travel purpose (business or leisure). Such data can also reveal ideas about new benefits to recommend to insurance companies that yield more useful products for travelers.
Over the course of time, his team has helped make travel insurance more transparent and easier to understand for travelers.
Changing Roles
Fezatte initially was unaware of jobs outside of the insurance industry. Like others, he found his job through a recruiter. When he learned of the position, he was attracted by the opportunity to establish new processes and procedures from the ground up while having the leeway to innovate new solutions. “Don’t be afraid of titles that do not say actuary,” he advises other actuaries. “There are plenty of interesting roles out there that are directly related to risk.”
Reducing Risk from Infectious Disease
Cathine Lam, Data Scientist, Economics & Actuarial Team, Metabiota
Cathine Lam’s passion to minimize the social and economic consequences of widespread infectious diseases explains why she joined Metabiota more than a year ago.
Seeing the Severe Acute Respiratory Syndrome (SARS) virus halt business operations and travel in Hong Kong and other parts of Asia about 15 years ago left a lasting impression on Lam. To prevent future outbreaks, she says, countries with severe outbreak history still scan entrants for fever or flu-like symptoms.
Lam worked for Milliman as an actuarial consultant for eight years. She hopes to “relieve the financial burden of recovery” in her work as part of Metabiota’s economics and actuarial team. Metabiota supports and develops products and services that help track and anticipate the social and economic repercussions of pathogenic microbial agents. The company’s clients include government agencies, businesses, reinsurers and insurers.
As the company’s sole property-casualty actuary, one of her primary responsibilities involves developing and enhancing the infectious disease stochastic catalog. The catalog offers millions of hypothetical, scientifically plausible simulations to fill in the gaps from historical outbreak data.
For disease research in academia, infectious disease spread models have developed significantly, Lam explains, but there is still work to be done on economic cost impact. “My role in the team is producing realistic modeled loss cost outcomes, which ultimately produces metrics that could be used by our clients to develop coverage or policy planning,” she says.
Hundreds of biological, socioeconomic, political and environmental data sources are fed into the models, including pathogen characteristics, country preparedness and global travel patterns. Historical outbreak data and Metabiota’s infectious disease stochastic catalog are accessible for clients through a multifunctional dashboard.
Seeing the SARS virus halt business operations and travel in Hong Kong and other parts of Asia . . . left a lasting impression on Lam.
Historical data includes active tracking of diseases including influenza, Zika, measles, Middle East Respiratory Syndrome (MERS) and cholera by location and pattern. The stochastic catalog simulates risk impacts and interventions by population or policy exposure.
Besides helping government agencies prepare to minimize the spread and risk of potentially life-threatening pathogens, Metabiota offers reinsurers and insurers assistance as well. The company’s dashboard provides modeled loss outcome by pathogens and event types as well as the thresholds for parametric triggers by location and disease type for insurers that want to provide coverage for their portfolio. Lam also works on product development. One current project is developing coverage for business interruption due to disease.
The Actuarial Advantage
Applying her actuarial mindset and the Actuarial Standards of Practice (ASOPs) benefits Lam’s efforts. Effective communication, data quality evalutation when data is sparse and the appropriate treatment of catastrophe losses are just some of the ASOP applications that contribute to more reliable results, she says. Her actuarial background also provides a unique perspective for creating actionable and actuarially sound metrics to estimate infectious disease risk.
Because she has also held internships at noninsurance entities, such as IBM, Lam is comfortable applying her actuarial skills in her current role. She says that when serving in a new industry, it is important for actuaries to “have an open mindset because there will be a lot to learn.” Lam recognizes that getting outside the zone of traditional actuarial positions could be uncomfortable, but she encourages actuaries to do it anyway. “It will be rewarding when you use your cross-industry analytical and actuarial skills to help solve problems,” she says.
As for working on a multidisciplinary data science team, Lam says it is important to “understand that professionals from other industries can offer different insights.” Actuaries should also embrace big data and technology, she advises. “Think of them as tools that can help you along the way.”
Accessing Risk and Safety With Granular Intensity
Frank Chang, Director of Insurance and Safety Analytics, Uber Technologies Inc.
When Frank Chang joined Uber Technologies Inc. as its lead actuary three years ago, he faced a tricky proposition: how to ensure peer-to-peer drivers have coverage beyond the limits and exclusions of their personal auto policies.
The company was already contracting with insurers willing to provide coverage by the mile, but it needed to better understand the right price for this coverage. Since jurisdictions differ on requirements and personal auto insurance policies vary by limits and language, his task was to estimate the cost of insurance at every stage of a trip — from going to pick up clients, transporting them and returning home.
To monitor the cost per mile, Chang’s team collects detailed information through the Uber app, which includes GPS, accelerometer and gyroscope information to glean insight on velocity, location, time of day, hard brakes and accelerations.
That was quite a challenge when purchasing auto insurance by the mile was as new as the introduction of Uber’s peer-to-peer transportation services. For state insurance and transportation regulators, this was new territory as well.
Before Uber ventured into peer-to-peer driving services, the company provided livery and “black car” services covered by commercial auto insurance. When the company chose to advance into peer-to-peer transportation, its drivers, who generally work part time on an as-available basis, found commercial auto insurance cost-prohibitive.
Uber’s peer-to-peer drivers do not buy commercial insurance coverage. Instead, the company purchases commercial coverage from insurers and retains part of the losses. Since Uber keeps some loss, Chang’s department applies analytics to manage risk and to assure appropriate reserving in the United States and worldwide.
“The core of what we do is reserving at a very granular accurate level,” he says. Due to the amount of exposure and the potential costs of miscalculation, “the need for accuracy at Uber is very high.”
The Route to Uber
Chang’s path to actuarial work began after he finished his Ph.D. in mathematics. At that time, he was a stay-at-home dad changing diapers and looking for flexible part-time work. He found it by being an equities analyst for The Motley Fool and teaching piano. His evaluation of insurance stocks introduced him to the idea of becoming an actuary.
He began taking two actuarial exams at a time while still being a stay-at-home dad. When his second son reached 9 months old, Esurance Car Insurance hired him as an actuarial analyst. He finished the exam process while serving as an actuarial analyst for Firemen’s Fund Insurance. For four years, his actuarial work ran the gamut of nearly every line the insurer offers — from reserving to reinsurance to commercial admitted and non-admitted insurance.
“That is what allowed me to be ready for my time at Google,” he recalls. At the time, Google was seeking an in-house actuary to save on consulting fees and to complete other projects. The company had three goals for Chang: first, to calculate reserves for the company’s captive and to estimate costs for a few dozen lines of retained loss; second, to price warranties in 2012 when Google was entering the consumer electronics market; and third, to help establish a Google credit risk model for the company’s AdWord customers.
“I finished most of my responsibilities in the first few months and my manager needed to find more work for me,” he recalls. After pursuing other projects, such as enterprise risk models and fraud, he was ready for a new challenge. So he contacted one of his former Google colleagues, the first chief financial officer for Uber, and became an in-house actuary there in 2014.
While solving the tricky proposition of producing very granular and per-mile loss estimates, he built a 22-employee team of actuaries, data scientists and modelers. Currently, he is expanding his department to include more actuaries.
“Our risk covers billions of miles in the U.S. alone each year,” he said. “Being able to scale insurance analysis globally has issues galore.” Uber operates in dozens of countries internationally, so third-party motor liability, passenger accident, driver accident and other coverages are part of the mix.
“Uber”-granularity
Estimating potential losses as accurately as possible requires going ultra-granular, he says. To monitor the cost per mile, Chang’s team collects detailed information through the Uber app, which includes GPS, accelerometer and gyroscope information to glean insight on velocity, location, time of day, hard brakes and accelerations.
This extra data allows more accurate risk assessment. In traditional insurance, a driver could report their residence as Philadelphia when they mostly drive in Manhattan. “Rating on garage and zip code screws up your model because you don’t really know what the true exposure is,” he observes.
To improve risk management, Chang also manages Uber’s safety data science team, which determines how to make the platform safer for everyone. The team uses telematics data, such as driving style, to encourage drivers to reduce harsh braking “because riders complain about hard brakes!” he says. Since Uber drivers are legally independent contractors, they can travel as they wish. However, Uber does provide “nudges,” such as app banners, to remind drivers when they are going too fast. The safety research team is also looking at how to mitigate interpersonal conflicts and encourage safety.
Encouraging Actuarial Success
For actuaries who want to move into new roles, he suggests they learn as much as possible about insurance because “you become the insurance expert.” He also believes actuaries should broaden their horizons. “We cannot think of ourselves as just actuaries — We need to think of ourselves as broad consultants; we need to be able to handle large datasets and have a data science toolkit. We also need to be able to understand how insurance works, what is in a policy and how is insurance is transacted.”
Chang is seeking actuaries who are “intellectually curious” and comfortable with trying new approaches. After interviewing nearly 1,000 actuaries and actuarial analysts, he finds that most want to work within what they know. For example, some applicants want to start with clean data, saying, “I only want to square triangles” or want a very routine role. While that is expected at insurance companies, Chang observes that nontraditional roles require applicants who want to work in a creative environment.
Annmarie Geddes Baribeau has been covering actuarial topics for more than 25 years. Her blog can be found at www.insurancecommunicators.com.